Correlational methods of research
Correlational Research: What it is with Examples
Our minds can do some brilliant things. For example, it can memorize the jingle of a pizza truck. The louder the jingle, the closer the pizza truck is to us. Who taught us that? Nobody! We relied on our understanding and came to a conclusion. We don’t stop there, do we? If there are multiple pizza trucks in the area and each one has a different jingle, we would memorize it all and relate the jingle to its pizza truck.
This is what correlational research precisely is, establishing a relationship between two variables, “jingle” and “distance of the truck” in this particular example. The correlational study looks for variables that seem to interact with each other. When you see one variable changing, you have a fair idea of how the other variable will change.
What is Correlational research?Correlational research is a type of non-experimental research method in which a researcher measures two variables and understands and assesses the statistical relationship between them with no influence from any extraneous variable.
Gather research insights
Correlational research ExampleThe correlation coefficient shows the correlation between two variables (A correlation coefficient is a statistical measure that calculates the strength of the relationship between two variables), a value measured between -1 and +1. When the correlation coefficient is close to +1, there is a positive correlation between the two variables. If the value is relative to -1, there is a negative correlation between the two variables. When the value is close to zero, then there is no relationship between the two variables.
Let us take an example to understand correlational research.
Consider hypothetically; a researcher is studying a correlation between cancer and marriage. In this study, there are two variables: disease and marriage. Let us say marriage has a negative association with cancer. This means that married people are less likely to develop cancer.
However, this doesn’t necessarily mean that marriage directly avoids cancer. In correlational research, it is not possible to establish the fact, what causes what. It is a misconception that a correlational study involves two quantitative variables. However, the reality is two variables are measured, but neither is changed. This is true independent of whether the variables are quantitative or categorical.
Mainly three types of correlational research have been identified:
1. Positive correlation: A positive relationship between two variables is when an increase in one variable leads to a rise in the other variable. A decrease in one variable will see a reduction in the other variable. For example, the amount of money a person has might positively correlate with the number of cars the person owns.
2. Negative correlation: A negative correlation is quite literally the opposite of a positive relationship. If there is an increase in one variable, the second variable will show a decrease and vice versa.
For example, being educated might negatively correlate with the crime rate when an increase in one variable leads to a decrease in another and vice versa. If a country’s education level is improved, it can lower crime rates. Please note that this doesn’t mean that lack of education leads to crimes. It only means that a lack of education and crime is believed to have a common reason – poverty.
3. No correlation: There is no correlation between the two variables in this third type. A change in one variable may not necessarily see a difference in the other variable. For example, being a millionaire and happiness are not correlated. An increase in money doesn’t lead to happiness.
Characteristics of correlational researchCorrelational research has three main characteristics. They are:
- Non-experimental: Correlational study is non-experimental. It means that researchers need not manipulate variables with a scientific methodology to either agree or disagree with a hypothesis.
The researcher only measures and observes the relationship between the variables without altering them or subjecting them to external conditioning.
- Backward-looking: Correlational research only looks back at historical data and observes events in the past. Researchers use it to measure and spot historical patterns between two variables. A correlational study may show a positive relationship between two variables, but this can change in the future.
- Dynamic: The patterns between two variables from correlational research are never constant and are always changing. Two variables having negative correlation research in the past can have a positive correlation relationship in the future due to various factors.
Gather research insights
Data collectionThe distinctive feature of correlational research is that the researcher can’t manipulate either of the variable involved. It doesn’t matter how or where the variables are measured.
A researcher could observe participants in a closed environment or a public setting.
Researchers use two data collection methods to collect information in correlational research.
Naturalistic observation
Naturalistic observation is a way of data collection in which people’s behavioral targeting is observed in their natural environment, in which they typically exist. This method is a type of field research. It could mean a researcher might be observing people in a grocery store, at the cinema, playground, or in similar places.
Researchers who are usually involved in this type of data collection make observations as unobtrusively as possible so that the participants involved in the study are not aware that they are being observed else they might deviate from being their natural self.
Ethically this method is acceptable if the participants remain anonymous, and if the study is conducted in a public setting, a place where people would not normally expect complete privacy. As mentioned previously, taking an example of the grocery store where people can be observed while collecting an item from the aisle and putting in the shopping bags. This is ethically acceptable, which is why most researchers choose public settings for recording their observations. This data collection method could be both qualitative or quantitative.
Archival data
Another approach to correlational data is the use of archival data. Archival information is the data that has been previously collected by doing similar kinds of research. Archival data is usually made available through primary research.
In contrast to naturalistic observation, the information collected through archived data can be pretty straightforward. For example, counting the number of people named Richard in the various states of America based on social security records is relatively short.
Use the correlational research method to conduct a correlational study and measure the statistical relationship between two variables. Uncover the insights that matter the most. Use QuestionPro’s research platform to uncover complex insights that can propel your business to the forefront of your industry.
Research to make better decisions. Start a free trial today. No credit card required.
SHARE THIS ARTICLE:
Correlational Research | When & How to Use
Published on July 7, 2021 by Pritha Bhandari. Revised on December 5, 2022.
A correlational research design investigates relationships between variables without the researcher controlling or manipulating any of them.
A correlation reflects the strength and/or direction of the relationship between two (or more) variables. The direction of a correlation can be either positive or negative.
Positive correlation | Both variables change in the same direction | As height increases, weight also increases |
---|---|---|
Negative correlation | The variables change in opposite directions | As coffee consumption increases, tiredness decreases |
Zero correlation | There is no relationship between the variables | Coffee consumption is not correlated with height |
Table of contents
- Correlational vs.
experimental research
- When to use correlational research
- How to collect correlational data
- How to analyze correlational data
- Correlation and causation
- Frequently asked questions about correlational research
Correlational vs. experimental research
Correlational and experimental research both use quantitative methods to investigate relationships between variables. But there are important differences in data collection methods and the types of conclusions you can draw.
Correlational research | Experimental research | |
---|---|---|
Purpose | Used to test strength of association between variables | Used to test cause-and-effect relationships between variables |
Variables | Variables are only observed with no manipulation or intervention by researchers | An independent variable is manipulated and a dependent variable is observed |
Control | Limited control is used, so other variables may play a role in the relationship | Extraneous variables are controlled so that they can’t impact your variables of interest |
Validity | High external validity: you can confidently generalize your conclusions to other populations or settings | High internal validity: you can confidently draw conclusions about causation |
When to use correlational research
Correlational research is ideal for gathering data quickly from natural settings. That helps you generalize your findings to real-life situations in an externally valid way.
There are a few situations where correlational research is an appropriate choice.
To investigate non-causal relationships
You want to find out if there is an association between two variables, but you don’t expect to find a causal relationship between them.
Correlational research can provide insights into complex real-world relationships, helping researchers develop theories and make predictions.
ExampleYou want to know if there is any correlation between the number of children people have and which political party they vote for. You don’t think having more children causes people to vote differently—it’s more likely that both are influenced by other variables such as age, religion, ideology and socioeconomic status. But a strong correlation could be useful for making predictions about voting patterns.To explore causal relationships between variables
You think there is a causal relationship between two variables, but it is impractical, unethical, or too costly to conduct experimental research that manipulates one of the variables.
Correlational research can provide initial indications or additional support for theories about causal relationships.
ExampleYou want to investigate whether greenhouse gas emissions cause global warming. It is not practically possible to do an experiment that controls global emissions over time, but through observation and analysis you can show a strong correlation that supports the theory.To test new measurement tools
You have developed a new instrument for measuring your variable, and you need to test its reliability or validity.
Correlational research can be used to assess whether a tool consistently or accurately captures the concept it aims to measure.
ExampleYou develop a new scale to measure loneliness in young children based on anecdotal evidence during lockdowns. To validate this scale, you need to test whether it’s actually measuring loneliness. You collect data on loneliness using three different measures, including the new scale, and test the degrees of correlations between the different measurements.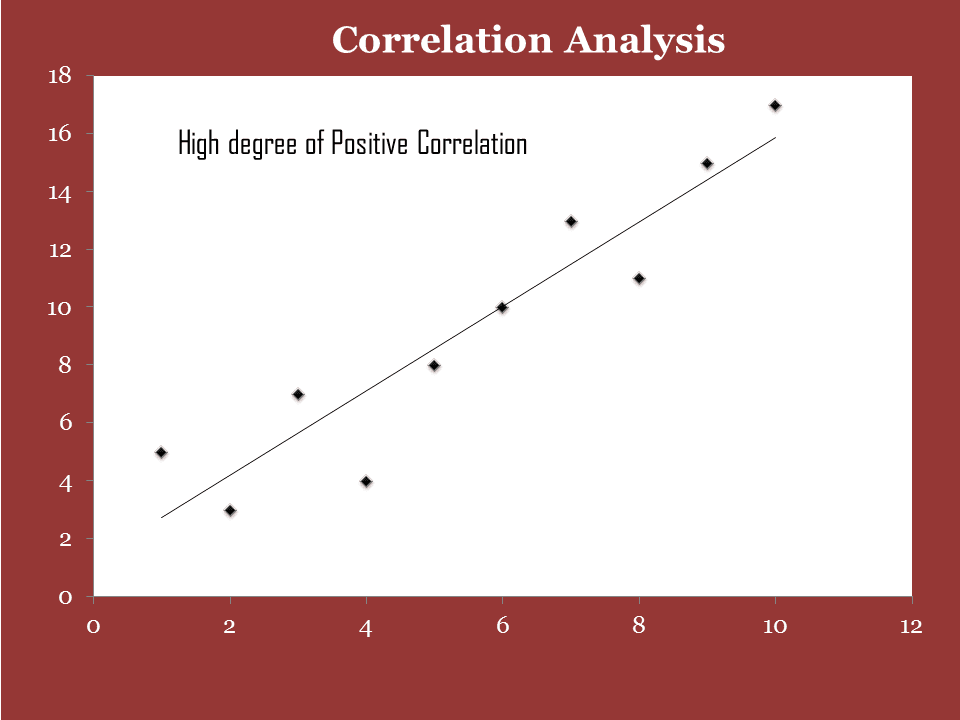
Prevent plagiarism. Run a free check.
Try for freeHow to collect correlational data
There are many different methods you can use in correlational research. In the social and behavioral sciences, the most common data collection methods for this type of research include surveys, observations, and secondary data.
It’s important to carefully choose and plan your methods to ensure the reliability and validity of your results. You should carefully select a representative sample so that your data reflects the population you’re interested in without research bias.
Surveys
In survey research, you can use questionnaires to measure your variables of interest. You can conduct surveys online, by mail, by phone, or in person.
Surveys are a quick, flexible way to collect standardized data from many participants, but it’s important to ensure that your questions are worded in an unbiased way and capture relevant insights.
Naturalistic observation
Naturalistic observation is a type of field research where you gather data about a behavior or phenomenon in its natural environment.
This method often involves recording, counting, describing, and categorizing actions and events. Naturalistic observation can include both qualitative and quantitative elements, but to assess correlation, you collect data that can be analyzed quantitatively (e.g., frequencies, durations, scales, and amounts).
Naturalistic observation lets you easily generalize your results to real world contexts, and you can study experiences that aren’t replicable in lab settings. But data analysis can be time-consuming and unpredictable, and researcher bias may skew the interpretations.
Secondary data
Instead of collecting original data, you can also use data that has already been collected for a different purpose, such as official records, polls, or previous studies.
Using secondary data is inexpensive and fast, because data collection is complete. However, the data may be unreliable, incomplete or not entirely relevant, and you have no control over the reliability or validity of the data collection procedures.
ExampleTo find out if working hours are related to mental health, you use official national statistics and scientific studies from several different countries to combine data on average working hours and rates of mental illness.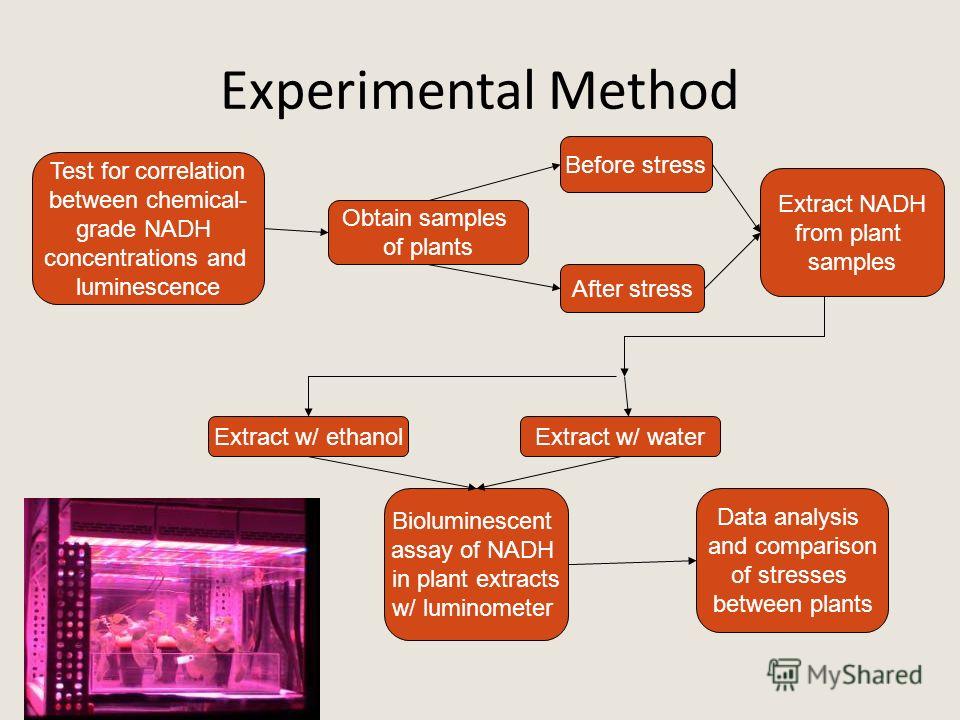
How to analyze correlational data
After collecting data, you can statistically analyze the relationship between variables using correlation or regression analyses, or both. You can also visualize the relationships between variables with a scatterplot.
Different types of correlation coefficients and regression analyses are appropriate for your data based on their levels of measurement and distributions.
Correlation analysis
Using a correlation analysis, you can summarize the relationship between variables into a correlation coefficient: a single number that describes the strength and direction of the relationship between variables. With this number, you’ll quantify the degree of the relationship between variables.
The Pearson product-moment correlation coefficient, also known as Pearson’s r, is commonly used for assessing a linear relationship between two quantitative variables.
Correlation coefficients are usually found for two variables at a time, but you can use a multiple correlation coefficient for three or more variables.
Regression analysis
With a regression analysis, you can predict how much a change in one variable will be associated with a change in the other variable. The result is a regression equation that describes the line on a graph of your variables.
You can use this equation to predict the value of one variable based on the given value(s) of the other variable(s). It’s best to perform a regression analysis after testing for a correlation between your variables.
Correlation and causation
It’s important to remember that correlation does not imply causation. Just because you find a correlation between two things doesn’t mean you can conclude one of them causes the other for a few reasons.
Directionality problem
If two variables are correlated, it could be because one of them is a cause and the other is an effect. But the correlational research design doesn’t allow you to infer which is which. To err on the side of caution, researchers don’t conclude causality from correlational studies.
Third variable problem
A confounding variable is a third variable that influences other variables to make them seem causally related even though they are not. Instead, there are separate causal links between the confounder and each variable.
In correlational research, there’s limited or no researcher control over extraneous variables. Even if you statistically control for some potential confounders, there may still be other hidden variables that disguise the relationship between your study variables.
There are many other variables that may influence both variables, such as average income, working conditions, and job insecurity. You might statistically control for these variables, but you can’t say for certain that lower working hours reduce stress because other variables may complicate the relationship.
Although a correlational study can’t demonstrate causation on its own, it can help you develop a causal hypothesis that’s tested in controlled experiments.
Frequently asked questions about correlational research
- What is a correlation?
-
A correlation reflects the strength and/or direction of the association between two or more variables.
- A positive correlation means that both variables change in the same direction.
- A negative correlation means that the variables change in opposite directions.
- A zero correlation means there’s no relationship between the variables.
- What’s the difference between correlational and experimental research?
-
Controlled experiments establish causality, whereas correlational studies only show associations between variables.
- In an experimental design, you manipulate an independent variable and measure its effect on a dependent variable.
Other variables are controlled so they can’t impact the results.
- In a correlational design, you measure variables without manipulating any of them. You can test whether your variables change together, but you can’t be sure that one variable caused a change in another.
In general, correlational research is high in external validity while experimental research is high in internal validity.
- In an experimental design, you manipulate an independent variable and measure its effect on a dependent variable.
Cite this Scribbr article
If you want to cite this source, you can copy and paste the citation or click the “Cite this Scribbr article” button to automatically add the citation to our free Citation Generator.
Bhandari, P. (2022, December 05). Correlational Research | When & How to Use. Scribbr. Retrieved March 22, 2023, from https://www.scribbr.com/methodology/correlational-research/
Cite this article
Is this article helpful?
You have already voted. Thanks :-) Your vote is saved :-) Processing your vote...
Pritha has an academic background in English, psychology and cognitive neuroscience. As an interdisciplinary researcher, she enjoys writing articles explaining tricky research concepts for students and academics.
Correlation study - Psychologos
Correlation analysis is a method of processing statistical data, which consists in studying the coefficients (correlations) between variables. In this case, the correlation coefficients between one pair or many pairs of features are compared to establish statistical relationships between them.
General information
The goal of correlation analysis is to provide some information about one variable with the help of another variable. In cases where it is possible to achieve the goal, the variables are said to be correlate . In the most general form, the adoption of the hypothesis of the presence of a correlation means that a change in the value of variable A will occur simultaneously with a proportional change in the value of B: if both variables increase, then the correlation is positive , if one variable grows and the second decreases the correlation is negative .
Restrictions
1. Application is possible if there are enough cases to study: for a particular type of correlation coefficient is from 25 to 100 pairs of observations.
2. The second limitation follows from the hypothesis of correlation analysis (see above), which includes a linear dependence of variables . In many cases, when it is reliably known that the dependence exists, the correlation analysis may not give results simply because the dependence is not linear (expressed, for example, as a parabola).
3. By itself, the fact of correlation does not give grounds to assert which of the variables precedes or is the cause of changes, or that the variables are generally causally related to each other, for example, due to the action of a third factor. (see Spurious Correlation, below).
Scope
This method of processing statistical data is very popular in the social sciences (especially in psychology), although the scope of correlation coefficients is extensive: quality control of industrial products, metallurgy, agrochemistry, and so on.
The popularity of the method is due to two points: the correlation coefficients are relatively easy to calculate, their application does not require special mathematical training. Combined with the ease of interpretation, the ease of application of the coefficient has led to its widespread use in the field of statistical data analysis.
False correlation
Often, the alluring simplicity of a correlation study encourages the researcher to draw false intuitive conclusions about the presence of a causal relationship between pairs of traits, while correlation coefficients establish only statistical relationships.
This is illustrated by a well-known anecdote: if you go outside and measure the size of shoes and IQ of 1000 random passers-by, a statistically significant correlation will be found between them. However, this does not mean that foot size affects intelligence, since factors such as gender and age of study participants most likely influenced the presence of this relationship.
In the modern quantitative methodology of the social sciences, there has actually been a rejection of attempts to establish causal relationships between observed variables by empirical methods. Therefore, when researchers in the social sciences talk about establishing relationships between the variables under study, they imply either a general theoretical assumption or a statistical dependence.
Correlation research in psychology
Correlation research in psychology is the most common form of experiment, due to a number of reasons. Firstly, researchers increasingly single out such complex phenomena as the subject of study that their functional control by organizing active experimental influences is impossible, difficult or undesirable. Secondly, many processes that are in principle accessible to experimental control lose their qualitative specificity if they are artificially isolated in a strict laboratory experiment. Thirdly, in a number of studies, for ethical reasons, the scientist cannot influence the variables under study. In these cases, the psychologist is content with the methods of observation and correlation research.
Correlation research is based on theoretical ideas about the measures of correlations between variables developed by K. Pearson. The strategy for conducting such a study is that there is no controlled impact on the object. The researcher puts forward a hypothesis about the presence of a statistical relationship between two (or more) variables. However, the assumption of causal dependence is not discussed.
The design of the correlation study is a kind of quasi-experimental design in the absence of the influence of the independent variable on the dependent ones.
The hypotheses tested using the correlation method are hypotheses about relationships. They claim that changes in one variable are somehow related to changes in another, but do not assume that any of these variables is causal.
Correlation is a study conducted to confirm or refute the hypothesis of a statistical relationship between several (two or more) variables.
![]()
For example, we could determine the correlation between the number of hours spent sleeping at night and daytime sleepiness. If the correlation is large, knowing how long a person sleeps at night will allow us to predict how sleepy they are during the daytime hours. In addition, daytime sleepiness can be used to predict the duration of nighttime sleep. Suppose a psychologist notices a relationship between the IQs of parents and their children, or between beauty and social popularity, or between anxiety and test scores, or between crime and the weather. In each case, two observations or events are correlated (related to each other in an ordered manner).
The strength and direction of the relationship can be expressed as a correlation coefficient. This is a number between +1.00 and -1.00. If this number is equal to or close to zero, there is little or no relationship between the two measured quantities. For example, the correlation between shoe size and intelligence is zero. If the correlation is +1.00, there is an absolute positive relationship; if it is -1.00, an absolute negative relationship is found.
Correlations in psychology are rarely absolute. But the closer the coefficient is to +1.00, the stronger the relationship. For example, identical twins tend to have nearly identical IQs. The IQs of parents and their children have only a general similarity. The correlation between the IQ of parents and children is 0.35; in identical twins, it is 0.86.
A positive correlation shows that an increase in one value is accompanied by an increase in another (or a decrease corresponds to a decrease).
For example, there is a positive correlation between high school performance and college performance; students who perform better in school tend to perform better in college (and vice versa).
If the correlation is negative , the increase in the first value is associated with a decrease in the second.
When talking about correlation, the terms "correlation" and "correlation" are used. A correlation is a consistent change in two or more features. Correlation dependence is the change that the values of one attribute make in the probability of occurrence of different values of another attribute. Both terms are often used interchangeably. Meanwhile, dependence means influence, connection - agreed changes. correlations cannot be considered as evidence of a causal relationship, they only indicate that changes in one feature, as a rule, are accompanied by certain changes in another. But whether the cause of the changes is in one of the signs or whether it is outside the pair of signs under study, we do not know.
We can speak in the strict sense of dependence only in those cases when we ourselves exert some kind of controlled influence on the subjects. If the study includes independent variables that we can at least take into account, for example, age, then we can consider the correlations revealed between age and psychological characteristics as correlation dependencies. In most cases, it is difficult for us to determine what is the independent variable in the considered pair of features and what is the dependent variable. Given that the term "dependence" implicitly or implicitly implies influence, it is better to use the more neutral term "correlation".
For example, we may notice that students who spend many hours in front of the TV get lower grades than those who devote a little time to this activity (the well-known “TV zombie” effect). Does this indicate that watching too much TV is causing poor academic performance? It is possible to make such an assumption, but we cannot be sure until we set up an experiment.
The presence of a correlation between two variables does not say anything about the cause-and-effect relationships between them, but it makes it possible to put forward such a hypothesis. The absence of correlation allows us to reject the hypothesis of a causal relationship of variables. There are several interpretations of the presence of a correlation between two measurements:
- Direct correlation.
The level of one variable directly corresponds to the level of another. An example is Hick's law: the speed of information processing is proportional to the logarithm of the number of alternatives. Another example: the correlation of high personal plasticity and a tendency to change social attitudes.
- Correlation due to 3rd variable. For example, the rate of image recognition during rapid (tachistoscopy) presentation and the subjects' vocabulary are positively correlated. The hidden variable behind this correlation is general intelligence.
- Random correlation not due to any variable.
- Correlation due to sample heterogeneity. Let us imagine that the sample that we will survey consists of two homogeneous groups. For example, we want to find out if belonging to a certain gender is associated with the level of extraversion. "Measuring" gender does not cause difficulties, but we measure extraversion using the Eysenck questionnaire. We have 2 groups: male mathematicians and female journalists.
Not surprisingly, if we get a linear relationship between gender and the level of extraversion - introversion: most men will be introverts, most women will be extroverts.
Correlations and causality. Correlation studies help us identify relationships and make predictions. However, correlation does not demonstrate causality (causation). For example, it may turn out that more time in front of the TV is spent by students who are not interested in the subjects they study. If this is the case, then both the lack of study effort and the lower grades are the result of disinterest, not excessive TV watching. The seeming connection of one thing with another does not mean that there is a causal relationship between them.
Consider the case where correlation can be mistaken for causality. Suppose a psychologist has determined that the blood of schizophrenic patients contains some chemical substance not found in healthy people. Does this mean that this substance is the cause of schizophrenia? It may seem that this is the case, but the formation of this substance can cause schizophrenia itself. Or the schizophrenia and the chemical may both be the result of some unknown factor, such as the diet in psychiatric hospitals.
Just because one thing appears to be the cause of another does not confirm that it is. This fact can be clearly seen in the example of some apparently non-causal connections. For example, there is a correlation between the number of churches in cities and the number of bars; the more churches, the more bars. Does this mean that drinking makes a person devout? Of course, in this case, no one would think of making such a conclusion about cause and effect. But in less obvious situations, the temptation is great. (The real relationship is that both the number of churches and the number of bars are related to the population of cities.) The best way to make sure there is a causal relationship is to run a controlled experiment.
The main thing that distinguishes the correlation approach is data collection schemes that are different from the experimental approach, and, accordingly, other possibilities for meaningful conclusions when testing psychological hypotheses (due to the impossibility of implementing those forms of control that are typical for experimental studies). With the correlation approach, the degree of arbitrariness of the meaningful interpretation, justified by certain statistical decisions, is much higher, at the same time, the conclusions are less conclusive, since if a significant relationship is established, there are many explanations (or theoretical hypotheses) regarding its nature and direction.
The main advantage of correlation analysis is that we can immediately perform multiple feature matching.
The task of any correlation study, as well as experimental, is generalization, i.e. dissemination of meaningful conclusions about the studied dependence in a wider context of understanding than limited by the framework of given situations, populations, variables, etc. However, the correlation approach always preserves the ambiguity of the conclusions from the point of view of the directions of possible generalizations. The limitations of control in obtaining empirical data also determine the limitations of admissible conclusions.