How is psychology different from common sense
Difference Between Psychology and Common Sense
Key Difference – Psychology vs Common SensePsychology and common sense refer to two different things between which a key difference can be identified. First, let us define the two words. Psychology refers to the scientific study of the mental processes and behavior of the human being. On the other hand, common sense refers to good sense in practical matters. As you can see the key difference between psychology and common sense, stem from its source of knowledge. Psychology relies on science, theoretical understanding, and research, but common sense relies on experience and reasoning . This is the main difference between the two words. Through this article let us attempt to gain a clearer understanding of the two words.
What is Psychology?
Psychology refers to the scientific study of the mental processes and behavior of the human being. Psychology is a broad field of study that consists of various subfields such as social psychology, abnormal psychology, child psychology, developmental psychology, etc. In each of these branches, attention is paid to the individual’s mental processes or mental health.
One of the key characteristics of psychology is that it focuses on the individual rather than on the group. Even when conducting experiments the individual is at the center. Also, Psychology is a discipline that has an extensive theoretical background with many theoretical perspectives such as Functionalists perspective, Cognitive perspective, Behaviorists perspective, Humanistic perspective, etc. Each perspective allows us to look at the human being in different ways. For example while behaviorists emphasize the importance of human behavior in Psychology, Cognitive theorists focus on the cognitive processes.
As you can see, psychology is a scientific field that relies on theory and experiments, but when you look at common sense, you will realize that there is a vast gap between psychology and common sense. In order to realize this now let us look at common sense.
What is Common Sense?
Common sense refers to good sense in practical matters. This is something that is essential for people when engaging in daily activities. Common sense allows people to be practical and reasonable. It makes them arrive at conclusions or make decisions based on the experience that they have.
The lay person usually is devoid of scientific knowledge; hence this role of knowledge is fulfilled by common sense as it directs the person to arrive at sound judgments in life. You may have heard people blaming others for not having common sense, in such situations the individual is referring to the lack of practical everyday knowledge.
Aristotle spoke of common sense.
What is the difference between Psychology and Common Sense?
Definitions of Psychology and Common Sense:
Psychology: Psychology refers to the scientific study of the mental processes and behavior of the human being.
Common Sense: Common sense refers to good sense in practical matters.
Characteristics of Psychology and Common Sense:
Scientific:
Psychology: Psychology is a field of study that is scientific.
Common Sense: Common sense is not scientific, but based on reason.
Branch of study:
Psychology: Psychology is a discipline.
Common Sense: Common sense is not a discipline.
Conclusions:
Psychology: In Psychology we arrive at conclusions through research or experiments.
Common Sense: When speaking of common sense, we use previous experience.
Theoretical Standing:
Psychology: Psychology has a clear theoretical basis.
Common Sense: Common sense does not have a theoretical basis.
Image Courtesy:
1. Positive Psychology Optimism by Nevit Dilmen [CC BY-SA 3.0 or GFDL], via Wikimedia Commons
2. “Aristotle Altemps Inv8575” by Copy of Lysippus – Jastrow (2006). [Public Domain] via Commons
Common Sense Versus Psychology: Explained With Examples
by Pragati Kalive
Psychology and Common Sense: Psychology can be understood as the science of human behaviour that seeks to understand how human beings feel, perceive, think, learn, interact and understand themselves and the world around them. It is a science that studies observable and measurable human behaviour and also the human mind, both the conscious and the unconscious. Since all of us as individuals are constantly attempting to understand each other’s actions, motivations and attitudes, there is a tendency to dismiss psychology as common sense. While psychology is a science that derives its assumptions and theories based on scientific knowledge that is generated from research and experiments, common sense remains a term given to shared beliefs or notions about the social and physical world that are not formulated through systematic testing.
It may range from routine knowledge about the world such as ‘sugar is sweet’ and ‘grass is green’, to common sense perceptions such as stereotypes and prejudices that are a result of generalisation. Although unscientific, common sense is based on reason and is therefore elevated to a position of high value, which leads some people to believe that there is no difference between common sense and psychology. However, unlike psychology, common sense has no theoretical basis and is solely based on past human experience which can truly be misleading when arriving at conclusions.
The assumptions of common sense often lie in stark contrast to the findings of psychological research. An example that highlights such incongruence is the infamous Milgram Experiment that was conducted by Stanley Milgram of Yale University. It was a series of social-psychological experiments that began in 1961 and sought to study obedience. The 40 male participants were assigned either the role of a ‘teacher’ or a ‘learner’. The teachers had to conduct memory tests for the learners. For every wrong answer, the teacher would have to administer an electric shock to the learner using a button. After every wrong answer, the intensity of the shock would be increased by 15 volts up to a maximum of 450 volts. What was not known to the teachers was that the learners would not receive any shock and all the ‘learners’ were actually actors who would convey the pain and trauma of the supposed shock through their acting. Before conducting the experiment, Milgram reached out to experts and amateurs to predict the result of the experiment, all of who had a similar prediction; virtually nobody would administer an electric shock of 450 volts for failing a memory test. To their surprise, Milgram’s experiment revealed that, on average, nearly 50% of the participants would go as far as administering the maximum punishment (“Differences between psychology and common sense”, 2015).
The lesson here is that despite the shared assumption that almost nobody would be willing to torture someone for giving incorrect answers on a memory test, especially since such actions would cause great internal conflict with an individual’s personal conscience, close to half of the participants in a scientific study obeyed the directions of the experiment. As opposed to assumptions of common sense, through this experiment, we can draw inferences about human behaviour that can be definitively applied in other contexts.
The problem posed by excessive reliance on common sense is that it provides us with a false sense of understanding of social and physical environments. However, the study of psychology challenges our assumptions and preconceived notions to find scientific answers to the questions posed by peculiar occurrences in our surroundings.
Importance of Common SenseThis is not to say that common sense is an entirely useless element of our cognitive processes. It aids us in making practical decisions by accounting for the potential consequences of our actions. It also becomes preferable to surround ourselves with individuals who subscribe to the same set of common-sense beliefs as us. Common sense can be considered a valuable resource as it becomes virtually impossible to process and function without certain essential common-sense ideas. It is common sense to look both ways before crossing the road, to turn off the gas after we finish cooking and not to approach wild animals. It is a sense that is cultivated over time and is therefore prized.
As social beings that are born, raised and shaped by culture, we must also understand common sense as a cultural sense that refers to a shared set of beliefs that owe their roots to the culture they originate from. For example, while it may seem evident that violence against women is a deplorable act, some cultures make allowances for certain situations wherein such actions are justified. Similarly, a considerable amount of the population subscribes to the idea that money is a source of contentment because abundance makes people happy. In contrast, another section of human beings recognise money as a source of tyrannical power and, therefore, alternatively subscribe to more socialistic ideas. On the other hand, research finds that the power of gratification possessed by money becomes extinct beyond a certain threshold. The cited examples are, in fact, a set of shared cultural beliefs that fall under the umbrella of common sense. These are important to acknowledge because such variations can assist behavioural and social psychologists in conducting better research. As a discipline, psychology also varies cross-culturally and is therefore similar to common sense in that respect. The difference, however, is that while common sense considers the beliefs, psychology analyses them to find the influences that shape such views, the consequences of assenting to such belief systems and how such beliefs and attitudes can be altered. We can, therefore, look at common sense as a resource that psychologists can tap into so that they may derive scientific knowledge out of it.
The practicality of common sense, although attractive, must be viewed with caution because of its potential to be misleading. While we acknowledge the shortcomings of common sense, recognising it as an asset to understanding more complex ideas is pertinent. Common sense and psychology must work in tandem in order to generate new bodies of relevant knowledge.
References
Differences between psychology and common sense. UKEssays.com. (2015). https://www.ukessays.com/essays/psychology/differences-between-psychology-and-common-sense-psychology-essay.php.
Fletcher, G. (1987). (PDF) Psychology and Common Sense. ResearchGate. https://www.researchgate.net/publication/3228869
Taylor, D. (n.d.). The importance of common sense. Amazing People. https://www.amazingpeople.co.uk/importance-common-sense/.
Categories PsychologyCopyright © 2023 The Sociology Group
From the psychology of common sense to the language of thinking. 12 Leading Modern Philosophers
From the psychology of common sense to the language of thinking
One of the two basic assumptions of the science of thought is that the mind is considered a machine that processes information. It is quite obvious that the mind really receives information from the surrounding world. Some of this information comes in the form of light waves acting on the retina of the eye, and some in the form of sound waves acting on the organ of hearing. Something else is also obvious: the way we act is not is dictated by from the information we receive. Different people, and even the same person, react differently to the same situations at different points in time. For example, there is no standard response to a sound wave pattern corresponding (in English) to the word "help". How we behave depends on what our mind does with the information it receives - on how it processes this information. If I run towards you when I hear you cry "Help!", then it is because my mind has somehow decoded your statement, identified it with an English word, understood what you want to say, and decided to react. All this is a very complex processing of information, which in the form of sound waves acted on my eardrum.
But how does this processing of information take place? How does the vibrations of the eardrum cause my muscles to contract, which is necessary to pull you out of the water and save you from drowning? Information must have carriers. We know how information is transmitted in the auditory system - the vibrations of the tympanic membrane enter the inner ear through the ossicular system. What happens next, as information is transmitted from the eardrum, is known less, but it is clear that an integral part of the overall picture of the mind as an information processor is the mandatory presence of physical structures that transmit information, that is, structures that are representations of the immediate environment (as well as, of course, things more abstract or remote). This is the second basic assumption of the science of thought. In essence, information processing is a matter of representational transformation, which leads to the activation of the nervous system, which gives you the order to jump into the water.
These are the two basic assumptions of the science of thinking:
1. Mind is an information processor.
2. Information processing involves the transformation of representations, which are physical structures for the transfer of information.
Fodor is particularly interested in those representations that correspond to desires, beliefs, and comparable psychological states, such as hopes and fears. In philosophy, they are usually called propositional relations, because they can all be described in the language of a thinker who is in various relations (for example, being in conviction or having hope) to private statements (for example, to statements that it is raining or that buses will start running soon. Fodor is convinced that there must also be internal representations corresponding to propositional relations. To some extent, and even quite often, we can explain and predict the behavior of other people, knowing what they think about the world and what they want to achieve. According to Fodor, the success of such explanations and predictions can only be explained by the fact that beliefs and desires actually drive our behavior. This means that beliefs and desires must have agents in internal structures by which they cause the bodily movements that we are trying to explain. Fodor calls this view intentional realism. This is a form of realism, because it associates propositional relationships with physical entities. And this realism is intentional, because propositional relations have a property that philosophers call purposefulness, that is, they proceed from the idea of a world arranged in a certain way.
What I call Fodor's "crown argument" is an argument leading from intentional realism to a special version of the computer model of the mind (which he himself calls a representational theory of mind). Intentional realism requires that we be able to think of beliefs and desires as things that can generate behavior. But this is a special case of causality. There is a fundamental difference between the movement of my leg when I'm trying to achieve something (like taking a 1,000-mile journey while taking the first step) and the movement of my leg caused by the impact of a neurologist's hammer under the kneecap. In the first case, what causes the movement is a desire, a purposeful desire, namely to make a journey of 1000 miles. This is what philosophers call content wishes. If the content changes, the form of movement will also change. Beliefs and desires become the causes of behavior by virtue of the fact that they reflect the idea of the world, that is, by virtue of their contents. Any satisfactory statement of intentional realism must explain how this type of action, which is caused by content, becomes possible. In particular, it is necessary to rationalize the relationship between beliefs and desires, on the one hand, and the behavior caused by them, on the other. Beliefs and desires condition the behavior that makes sense out of it.
The movement of the foot makes sense and is rational if I want to start a journey of 1000 miles and take a step in the right direction.
However, the causal relationship between behavior and content is extremely mysterious. In a sense, representations are simple objects, just like any other - they can be sound waves of a certain length, a collection of neurons, or sheets of paper. Thinking in this way, there is little difficulty in understanding how representations can cause certain behaviors. In fact, this causality is no different from the causation that causes the leg to twitch in response to a blow from a neurological hammer. But the whole point is that the representations that we are interested in - propositional relations - have a special0007 semantic relation to the world - that is, they make sense; they talk about something - for example, about someone's mother or about the planet Mars. The puzzle is not exactly how representations can cause behavior in the world, but how they do it—how they become functions of their meaning, functions of some relationship they have with other objects in the world (objects that , moreover, may not exist).
The great advantage of the computer model of the mind, according to Fodor, is that it helps solve the problem of causality due to content. In order to understand why we need to formulate this problem more clearly, Fodor, along with other specialists in the science of thinking, and also together with the vast majority of philosophers, adheres to the view that all manipulations performed by the brain with representations are purely physical and mechanical. . The brain and the representations it contains are physical entities, which means that they can only be sensitive to certain types of properties of mental representations. When I say the word "cat", I only produce a sound wave of a certain configuration. This sound wave has certain physical properties, due to which it affects the brain. Sound waves have amplitude, length, frequency and some other properties to which the brain is sensitive through the organs of hearing. But the fact that this sound conveys the idea of a cat for English-speaking people is a property of a completely different type. It is not a property to which the brain is directly sensitive (at least, that is Fodor's argument).
Let's call the physical properties that can be mechanically manipulated in the brain formal properties . We call them this because they refer to the physical form representation. And the properties by virtue of which representations represent meanings, we will call semantic properties, since semantics is a branch of linguistics that deals with the meaning of words (the way words represent the world). The fundamental source of the problem concerning the causal properties of content is semantic properties, not formal properties. It is easy to think of examples of pairs of representations that have the same formal properties but different semantic properties. Here, for example, two entries of numbers:
1101 1101
It is very easy to give a formal description of these two entries. Each can be described, for example, as two 1s followed by a 0, which in turn is followed by a 1. Or, more abstractly, we can describe them with vertical and horizontal dashes. It is clear, however, that the formal description of the left number will be identical to the formal description of the right one. However, it is quite possible that these formally identical entries may have completely different semantic properties. For example, the right number can be read as one thousand one hundred and one in the decimal number system, and the left one can be read as thirteen in the binary number system. Here we have two representations, identical in their formal and physical properties, but completely different in their semantic and semantic properties.
Thus, in this example, the problem of causality due to content clearly emerges. The brain is an information processing machine sensitive only to the formal properties of representations. As far as direct inputs to the brain are concerned, there is no difference between the left and right numbers. However, there is a big difference between the number 1101 and the number 13. Somehow our brain picks up on this difference. After all, we can still distinguish between 1101 as a binary number and 1101 as a decimal number. We see that these two records carry different information. Moreover, we are able to react differently to these two records. The problem of causality due to content is reduced to the problem of its possibility. How can the brain process information if it is blind to the semantic properties of representations? How can the brain be an information processing machine if all it can do is process the formal properties of representations?
In this regard, an important analogy can be drawn between the brain and a computer. Computers manipulate only sequences of characters, and we can think of these characters as representations that have a certain form. A computer programmed in binary, for example, manipulates sequences of 1s and 0s. The manipulations performed by the computer are determined solely by the shape of these characters. A sequence of ones and zeros can represent a natural number. For example, 10 is the number 2, and 11 is the number 3. However, the same sequences can represent completely different things. For example, the entry may indicate which of the pixels in a long row of pixels are on and which are off. Indeed, with the right encoding, almost anything can be represented with a sequence of 1s and 0s. However, from the point of view of a computer, it is completely indifferent what exactly the sequence of ones and zeros represents - that is, the semantic properties of this sequence are not important. The computer simply manipulates the formal properties of sequences of 1s and 0s.
Nevertheless, and this is very important, the computer is programmed to manipulate sequences of 1s and 0s in such a way that the correct result is obtained when interpreting, although the computer itself is blind in this respect and does not interpret. If a computer, for example, acts as a calculator and is given two sequences of ones and zeros, then it will simply calculate the third sequence. If the two sequences represent two numbers, 5 and 7, respectively, then the third sequence will be the binary representation of the number 12. But these semantic properties are irrelevant to the mechanics of the process performed by the computer. All the computer does is mechanically manipulate ones and zeros based on their formal properties. But at the same time, the computer is designed in such a way that these formal manipulations bring the desired result at the semantic level. The pocket calculator handles zeros and ones in such a way that it allows you to perform correct arithmetic operations with numbers represented by zeros and ones.
So, computers manipulate symbols based only on their formal properties, but in some way their semantic properties are also taken into account. This, Fodor argues, is exactly what the brain does. The brain is a physical system sensitive only to the formal properties of mental representations. But nevertheless, as a machine for processing information, the brain (like a computer) must take into account the semantic properties of mental representations. We can understand the application of Fodor's argument regarding intentional realism to the computer model of the mind as follows: since the brain and the computer must solve the same tasks (and we understand how the computer solves them), so the easiest way to understand how the brain works is is to think of the brain as a kind of computer.
But how exactly can one imagine the operation of such an analogy? The following three statements summarize Fodor's computer model:
1. Cause-and-effect relationships between content elements ultimately boil down to cause-and-effect relationships between physical states.
2. These physical states have a sentence structure, and their structure determines how they will be arranged and how they will interact with each other.
3. Causal transitions between sentences in the language of thought take into account meaningful relationships between the contents of these sentences in the language of thought.
The second and third statements express Fodor's contribution to the development of the problem of causal relationships between contents. Fodor's view is important, according to which the environment of thinking is what the philosopher calls the language of thinking. According to Fodor, we think in sentences, but they are not natural language sentences like English. The language of thought is more like a formal language, such as the predicate calculus developed by logicians to represent the logical structure of sentences and judgments in English. Like the languages with which computers are programmed - and the language of logic is in fact very close to them - the language of the predicate calculus seems to be free from the ambiguities and inaccuracies of the English language.
The analogy between the language of thought and computer languages and the language of predicate calculus is the core of Fodor's solution to the problem of causal relations between contents. This is what is implied in Statement #3. Fodor's basic condition that is common to formal languages is that formal and semantic properties must be clearly distinguished. From the point of view of syntax, a formal language - for example, the language of the predicate calculus - is simply a set of symbols of various types, together with rules for operating on these symbols, and these rules depend on the types of symbols. Symbols in the predicate calculus fall into several types. Some characters, usually represented by lowercase Latin letters (a, b, etc.), represent individual objects. Other characters, usually capital letters of the Latin alphabet, represent properties. Symbol combinations give an idea of the state of affairs. So, for example, if "a" represents Jane, and "F" the property "run", then the symbol "Fa" represents the position when Jane is running. The predicate calculus contains symbols corresponding to various logical operations, as, for example, the symbol "&" corresponds to a conjunction of symbols, and "-?" — character negation, the quantifiers "3" and "V" stand for "at least one" and "all", respectively.
The rules governing character combinations that form sentences can only be formulated in terms of the formal properties of individual characters. An example is the rule that a space after a capital letter (eg, the space "F-") can only be filled with a lowercase letter (eg, "a"). This rule at the syntactical level expresses the intuitive understanding that properties originally belong to things, but this in no way forces us to abandon the idea that uppercase letters are property names and lowercase letters are names of things. All of this is pure0007 syntax language. The application of a rule is a purely mechanical process of exactly the same type as the actions brilliantly performed by a computer.
On the other hand, the connection between the formal system and its content is manifested at the level of semantics. This happens when, in accordance with the semantics of a formal language, we attribute objects to certain constants, properties to predicates, and logical operators to relationships. To provide a language with semantics is to give an interpretation to the symbols contained in the language in order to turn the collection of meaningless symbols into a representational system.
Just as it is permissible to consider the symbols of a formal system from the point of view of syntax and from the point of view of semantics, transitions between symbols can be considered in the same way in two ways. The existential generalization rule in predicate calculus, for example, can be considered both syntactically and semantically. From a syntactic point of view, the rule allows that if in one line of the proof there is a formula of the form "Fa", then in the next line of the proof one can write the formula "3x Fx". Semantically speaking, the rule states that if it is true that something is "F", then it must also be true that something is "F" (because the interpretation of "3x Fx" says that there is at least one " F"). All transitions in a formal system can be viewed in these two ways—that is, either as rules for the manipulation of meaningless symbols, or as rules defining relationships between utterances to be interpreted.
Fodor's main suggestion is therefore to use the model of the relationship between syntax and semantics in the formal system to understand the relationship between a sentence in the language of thought and its content (what it represents and denotes). The mind is a computer that operates on sentences in the language of thought. These sentences can be viewed purely syntactically (as physical symbolic structures made up of basic symbols and arranged according to certain rules of composition) or semantically (based on how they reflect and represent the world, in which case they are viewed as some kind of judgments or statements. about the world). Developing this position, one can consider the transitions between sentences in the language of thought either syntactically or semantically: either in terms of formal relations that exist between physical symbolic structures, or in terms of semantic relations that exist between different representations of the world.
Let us return, however, to Fodor's third assertion. Information processing in the mind is ultimately the making of causal transitions between sentences in a language of thought, just as information processing in a computer is ultimately the making of causal transitions between sentences in a programming language. Let us assume that we proceed from the fact that causal transitions between sentences within the language of thought are purely formal, carried out by virtue of the formal properties of significant symbols, regardless of what these symbols stand for and what they refer to. In this case, the essence of our question boils down to the following: how are the formal relations that exist between sentences in the language of thought superimposed on the semantic relations that exist between the propositional judgments corresponding to these sentences? If we agree that the language of thought is a formal system, then this question can be answered quite clearly and unambiguously. Syntactic transitions between sentences in the language of thought will follow semantic transitions between the contents of those sentences, for exactly the same reasons that syntax follows semantics in any well-formed formal system.
Fodor can (and does) refer to the well-known data of metalogics (the study of expressive possibilities and the formal structure of logical systems), which made it possible to establish a significant degree of correspondence between syntactic derivability and semantic certainty. For example, it is known that the first-order predicate calculus is qualitative and complete. That is, it can be said that in every conscientiously executed proof in the first-order predicate calculus, the conclusion is indeed a logical consequence of the premises (quality). And vice versa, if for each argument, when using which the conclusion follows logically from the premise, the conclusion and the premise can be formulated in the language of the first-order predicate calculus, then the proof is qualitative (completeness). Using the terms we have introduced, we get: if a sequence of legitimate and formally defined transitional inferences leads from formulation A to formulation B, then we can be sure that A cannot be true if B is not true - and vice versa, if in the semantic sense A entails B, then you can be sure that there is a sequence of formally defined and derivable transitions leading from A to B.
Therefore, it can be said that beliefs and desires are expressed in terms of linguistic physical structures (sentences in the language of thought), and practical reasoning and other forms of thought must be interpreted in terms of causal interactions between these structures. These causal interactions are determined only by the formal, syntactic properties of physical structures. However, since the language of thought is a formal language that has analogues of the formal properties of quality and completeness, these purely syntactic transitions are made taking into account the semantic relations between the contents of relevant beliefs and desires. This is why (Fodor argues) content-driven causality takes place in such a purely physical system as the brain. So, Fodor continues, an adequate psychological interpretation is provided by the work of the mind, similar to the computer processing of sentences in the language of thinking.
From words to language
From words to language Those several words or concepts that are proposed here actually grow out of a single word and in themselves do not form a language, even the most concise one. These are only conditional outlines hinting at the possibility of language. Such that he was
THAT ALL PROBLEMS COME TO LANGUAGE
ABOUT ALL PROBLEMS REDUCED TO LANGUAGE The 1930s began with famine and ended in blood. Lines in front of public kitchens gave way to pickets outside factories and warehouses, which in turn were replaced by lines of soldiers leaving for the war. It has been convenient to operate for decades, although
9. ANTINOMIES OF MEANING
9. ANTINOMIES OF MEANING The categories that we will consider here are usually most often drawn on by aestheticians, since these are the most obvious, absolutely universal and primitively necessary categories. So, reasoning about "unity", "diversity",
Chapter 2. The Two Faces of Common Sense: An Argument for Common Sense Realism and Against Common Sense Theory of Knowledge{10}
Chapter 2 1. Apologia for Philosophy It is absolutely necessary these days to apologize for being interested in philosophy in any form. Except, be
Logic of meaning
Logic of meaning Foreword (from Lewis Carroll to the Stoics) Carroll's writings are always oriented towards the pleasure of the reader: children's books, or rather books for little girls; delightful strange esoteric words; ciphers and decryption;
Learning common sense
The study of common sense (I) "Another Latin work in which M. Descartes has gone far enough, and of which he has left us a rather large fragment, is the Study of Common Sense, or the Art of Right Thinking, which he called the Studium bonae
CHAPTER 3 PROBABLE CONCLUSIONS OF COMMON SENSE
CHAPTER 3 POSSIBLE CONCLUSIONS OF COMMON SENSE A "probable" conclusion (as we have already said) is such a conclusion in which the premises are true and the construction is correct, and the conclusion is nevertheless not certain, but only more or less probable. In the practice of science
"COMMON SENSE" PHILOSOPHY
PHILOSOPHY OF "COMMON SENSE" If Shaftesbury and Hutcheson criticized Locke's ethical views, then his sensualist philosophy in the 18th century was criticized by representatives of the so-called philosophy of common sense. Its first and most prominent representative was Thomas
Scientific enlightenment of common sense
Scientific enlightenment of common sense Naturally, common sense, which creates many illusions in its view of the world, must unreservedly allow the sciences to enlighten itself. But scientific theories invading the life world should not, in principle, touch the boundaries and spaces of our
§2. The categories of being and thinking of Shankara in comparison with the categories of being and thinking of Heidegger in the interpretation of J.
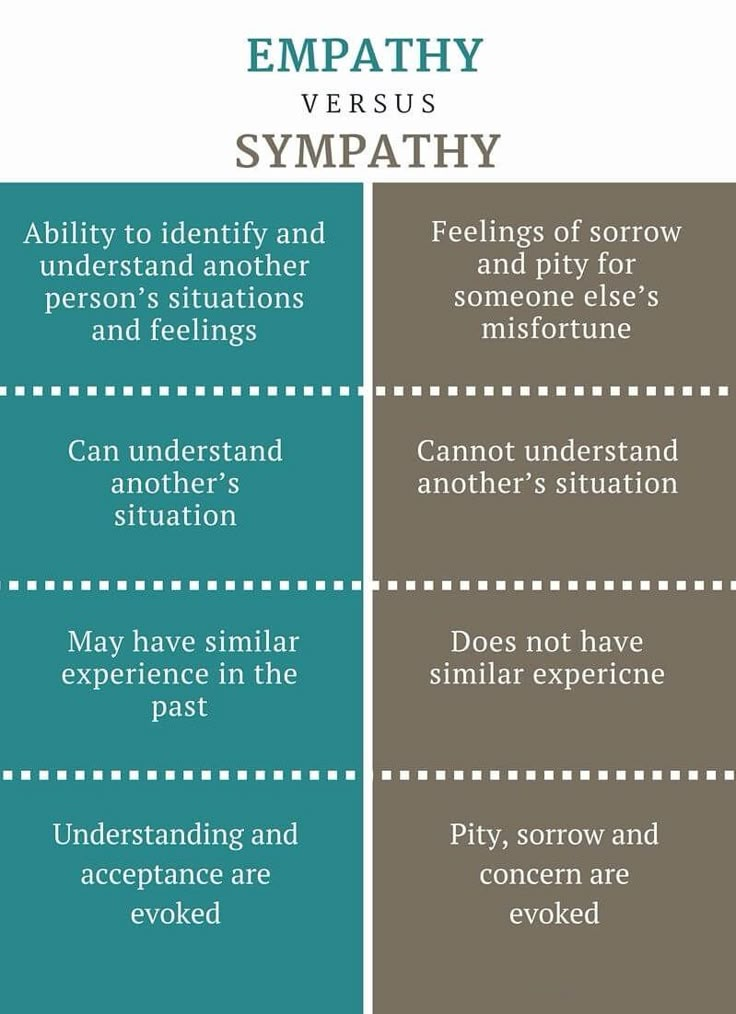
§2. The categories of being and thinking of Shankara in comparison with the categories of being and thinking of Heidegger in the interpretation of J. Mehta Modern Indian philosophers show quite a lot of interest in the work of Heidegger, and one of the most striking examples of this interest can be
Chapter Three Correlative Properties of Positive Thinking and Common Sense
Chapter Three Correlative Properties of Positive Thinking and Common Sense I. About the word "positive": its various meanings determine the properties of true philosophical thinking 30. A self-evident confluence of various general considerations given in this
COMMON SENSE ECONOMY
THE ECONOMY OF COMMON SENSE The essence of the coming economy, declares Stanford Research Institute's Long Term Planning Service, will be "an emphasis on the internal needs of the individual and group of people as much as on material needs. " This
XI. Meaning Leak
XI. Leakage of meaning The last cry of philosophical fashion, fortunately almost unheard in all-responsive Russia, has been called "new realism." The principles of the emerging doctrine were announced at a conference in Berlin, following which a collection of articles “Realism
assessment and comparison of the psychology of common sense / Sudo Null IT News
The curiosity of scientists is second only to the curiosity of children. When a child begins to speak, it is a great joy for parents. When the googoo-gaga is replaced by a completely intelligible speech, the flow of questions becomes almost endless. But even before this turning point in development, the child shows a keen interest in everything around him, especially in people. This interest is due to the process of self-learning. In particular, babies are able to analyze and understand what drives a particular person. In other words, infants are able to see the connection between a person, an object, and the task that the person is performing through that object. But artificial intelligence is not capable of such a trick, which was confirmed by scientists from New York University (USA). They conducted comparative experiments that assessed the so-called psychology of the common sense of infants and artificial intelligence. What kind of experiments were carried out, what did they show, and how superior are babies to AI? We will find answers to these questions in the report of scientists.
Study basis
The fundamental phenomenon that is studied in this work is folk psychology (or the psychology of common sense). This term refers to the totality of cognitive abilities, including the ability to predict and explain human behavior.
This ability is inherent not only in adults, but also in infants, who unconsciously try to understand the world around them as quickly and efficiently as possible. The process of early child development can sometimes feel incredibly fast and easy, especially in the context of learning about people, objects, and places. It is very difficult to train such a machine on the contrary. If the goal is to create AI that mimics a person as much as possible, then this difficulty must be overcome.
Scientists note that one of the main obstacles to creating AI based on common sense is the question - with what knowledge to start? The fundamental knowledge of the human infant is limited, abstract, and reflective of our evolutionary heritage, yet it can adapt to any context or culture in which that infant may develop. Therefore, to create a super cool AI, you need to start small, i.e., before creating an "adult" AI, you need to create its infant version.
Over the past few decades, fundamental research into the psychology of infant sanity, i.e., infants' understanding of the intentions, goals, preferences, and rationality underlying the actions of agents (people), has shown that infants attribute goals to agents and expect agents to achieve goals in a rational and efficient way. Predictions that support the psychology of infant sanity are at the heart of human social intelligence and thus could help improve AI sanity, but these predictions are typically missing from machine learning algorithms that instead predict actions directly and therefore lack flexibility. to new contexts and situations. However, research into the psychology of infant sanity has not yet been evaluated in a framework that can be directly tested against a machine.
Various descriptions of infant knowledge about agents suggest that this knowledge is:
- linked as a single set of abstract concepts of causal efficacy (cause-effect), efficiency, purposefulness, and perceptual access;
- reflect the infants' intuitive understanding of agents' mental states, which guides their effective actions in accordance with their mental states;
- or arise from individual achievements based on infants' own experience of action.
From this rich experimental and theoretical base emerges the need for a comprehensive framework in which infants' knowledge of agents could be characterized with comparable outcomes in one task to those in another, and comparable outcomes in a set of tasks for infants and machines.
In the work we are reviewing today, scientists demonstrate the basis they have developed for testing the psychology of common sense in infants by evaluating the results of infants in a test of children's intuition (BIB from Baby Intuitions Benchmark ) is a set of six tasks that explore the psychology of common sense. The BIB was designed specifically to test the intelligence of both infants and machines. A comparison was also made between infants and AI within the framework of common sense tasks.
Preparing for experiments
Image #1
The BIB tasks included short silent animation videos with simple visual effects (simple geometric shapes) performing basic movements within a space separated by a grid (image #1). This design allowed for the scalable procedural stimulus generation required for testing machine learning algorithms. This design also represented a new, overlay navigational context that required the assumption of full agent observability of the space and its contents.
It is worth noting that all BIB tasks were consistent with each other, which allowed different tasks to be compared without worrying about attributing null effects to different vision, memory, or other task requirements. Moreover, instead of focusing on a single principle of common-sense psychology, the BIB tasks focused on the three possible attributions that an observer can make of agents' actions - purpose attribution, rationality attribution, and instrumentality attribution. This helped to clarify whether such principles of common-sense psychology could be reconciled, and if so, how.
Attribution* is a mechanism for explaining the causes of another person's behavior.
Using the BIB environment, the scientists procedurally generated video stimuli to test infants and computational models and selected the most prominent examples of the specific principles of common-sense psychology targeted at each task.
The first three tasks focused on the observer's assignment of goals to the actions of agents.
The "goal" task reflects the idea that the goals of agents are directed to objects, not to places. Observers during familiarization watch as the agent repeatedly moves to the same one of the two objects at approximately the same place in the unchanged grid space. In testing, observers may be more surprised when the agent moves to a new object in that space after the positions of the two objects are swapped.
The multi-agent task asks if the targets are agent-specific. Observers watch the agent move towards the same one of two objects in a changing space, with both objects appearing in different places. In testing, observers may be more surprised when the original agent, rather than the new one, moves to a new object.
The unattainable goal problem asks whether agents can form new goals when their existing goals become unattainable. Observers watch the agent move towards the same one of two objects in a changing space, with both objects appearing in different places. When testing, the mesh space changes again so that the target agent becomes physically inaccessible. Observers may be more surprised when an agent jumps to a new object when its previous target is available, rather than vice versa.
The next two tasks focused on the observer's attribution of rationality to the actions of agents.
The "efficient agent" problem reflects the idea that agents act rationally to achieve goals. Observers watch as the agent moves efficiently towards the object, overcoming obstacles in an unchanging space. During testing, the object appears in the place where it appeared during familiarization, but the grid space changed in such a way that the obstacles that blocked the object disappeared or were replaced by other obstacles. Observers may be more surprised when the agent follows a familiar but already inefficient path towards the object.
The inefficient agent problem asks what observers expect from agents that initially move inefficiently in a changing space. During familiarization, observers watch how the agent moves towards the object along the same paths as the agent in the "efficient agent" problem, but this time there are no obstacles in the agent's path, so the agent's movements towards the object are inefficient. During testing, the environment changed, as in the "effective agent" task. Observers may either be more surprised when an agent continues to inefficiently move towards an object, or have no expectations as to whether that agent will move towards an object efficiently or inefficiently.
The last task focused on the observer's attribution of instrumentality to the actions of agents.
The instrumental action task reflects the idea that agents should only take instrumental actions when necessary. During familiarization, observers watch as the agent moves first to the key (tool) that he uses to remove the barrier around the object in different places, and then to this object. In testing, observers may be more surprised when the agent continues toward the key rather than directly toward the object when the barrier is no longer blocking the object.
All videos (stimuli) are available at this link.
The BIB task framework used the "wait violation" search time paradigm often used to test infants. Observers see a series of familiarization trials that serve to create an expectation followed by:
- an expected outcome that is perceptually different from familiarization, but conceptually consistent with it;
- an unexpected result that is perceptually similar to familiarization, but conceptually contradictory to it (therefore it surprises the observer).
Infant trials
Experiment #1 collected infant responses to two of the six BIB tasks (target and effective agent).
Mixed-model linear regressions assessed infant performance on each task, and an additional regression examined infants' overall performance on both tasks. Here the time of observation was the dependent variable, the outcome (expected and unexpected) was the fixed effect, and the participant was the intercept of the random effects. To obtain p-values, the scientists ran Type 3 Wald tests on the results of each regression.
Experiment #1 focused on these two tasks because the common sense they measured had consistent results in the literature on understanding infant actions. Thus Experiment #1 was intended to provide initial evidence for the psychology of common sense in infants.
Experiment #2 followed the pre-recorded design and analysis plan, repeating the two tasks in Experiment #1 with some improvements: automatic test progress; balancing the side of the target object between the participants of the "target" task; and matching durations of test trials among participants in the "effective agent" task. Infants were tested on these two tasks as well as on the other four BIB tasks described above that were not included in Experiment #1.
Experiment #1 included 11-month-old infants (N = 26, M age = 11.13 months, 12 girls and 14 boys) born at gestational age ≥37 weeks. They completed the goal task, the effective agent task, or both, with half of the babies completing the first task first. A total of 48 individual testing sessions and 24 sessions per task were conducted. An additional four sessions were excluded because the infants did not complete the session.
11-month-old infants (N = 58, M age = 11.06 months, 31 girls and 27 boys) born at gestational age ≥ 37 weeks. Each infant completed at least one BIB task for a total of N = 288 individual testing sessions.
All tests were conducted online via Zoom. Each trial video was preceded by a 5-second attention grab (a spinning spot accompanied by a ringing sound at the center of the screen) to focus the infant's attention on the screen, and each video would freeze after the agent reached the object. The last frame of the video remained on the screen until the infants looked away for 2 consecutive seconds or for 60 seconds.
Picture #2
Above are the results of the babies in the two tasks of experiment #1. The follow-up time in infants varied by task, with longer duration in the effective agent task compared to the target task, reflecting the longer duration of the test trial in the effective agent task. In general, infants looked longer at unexpected rather than expected outcomes.
Babies were surprised (watched longer) when the agent moved to a new object in the "target" task. The same picture was observed in the case when the efficient agent chose an inefficient path to the object in the "efficient agent" problem.
The results of the babies in experiment #2 are also shown in the graphs above. The follow-up time in infants varied by task, reflecting the different lengths of test runs for different tasks. In general, infants did not observe unexpected results longer than expected. But the outcome interaction task showed that different tasks elicited different gaze patterns in infants.
First, the results of the infants on the three tasks of Experiment #2 that focused on goal attribution were reviewed: the goal task, the multiple agents task, and the unattainable goal task. In accordance with the results of Experiment #1, the infants were surprised when the agent moved to a new object in the "target" task. In the case of the appearance of a new agent in the “multiple agents” task, the infants showed no difference in surprise when this agent moved to a new object compared to the original one. Babies in the "unreachable target" task also showed no difference in surprise when the agent moved to a new object when its target object was available versus unreachable.
The results of the infants were then examined in two tasks that focused on the attribution of rationality: the "efficient agent" and "inefficient agent" tasks. In accordance with the results of Experiment #1, infants were surprised when an efficient agent chose an inefficient path to an object in the "efficient agent" task. Infants in the "inefficient agent" task showed no difference in surprise when the agent continued to inefficiently move towards the object. As a result, there was no evidence that infants' surprise at a later failure of an effective agent was different from their surprise at a later failure of an effective agent.
In conclusion, the attribution of instrumentality of infants through their results in the task "instrumental action" was considered. The infants showed no difference in surprise when the agent moved towards the tool, as opposed to its target when the tool was no longer needed to reach the goal.
AI test
To find out if infants' knowledge of agents could be reflected in modern machine intelligence, the researchers compared the infants' performance on the BIB in Experiment #2 with the performance of three models of trained neural networks. The models formed predictions about the agent's actions during testing based on his actions during the familiarization. To obtain a continuous measure of surprise as a correlate of the infants' gaze time, the scientists calculated the models' prediction error for each frame of each result and considered the frame with the maximum error. Then, to compare the performance of the model and the infant, the average Z-score surprise score for each outcome for each model and the average Z-score search time for each outcome for infants were calculated. For an unplanned quantitative comparison of overall similarity between infant and each model scores, root mean squared error (RMSE) was estimated for the six BIB tasks using the mean Z-score for the unexpected result. There was also a comparison between infant performance and "baseline", which was assigned a "surprise score" of 0 across all tasks.
Because infants interact with stimuli such as the BIB through passive observation, the neural network models were based on the Theory of Mind Net (ToMnet) architecture, which is a neural network designed specifically for passive observation. It draws inferences about an agent's underlying mental states based on its behavior.
Image #3
Three models from two classes were tested with this architecture: behavioral cloning (BC from behavioral cloning ) and video modeling (diagrams above).
Two BC models predicted how an agent would act using background learning as examples of state-action pairs. To predict the agent's next action in a test trial, the BC model combined information from the learned features from the previous frame of the test video along with the learned features in the set of introductory videos. Video simulation used a similar strategy, architecture, and training procedure, but it aimed to predict the entire next frame of the test video, not just the agent's next action.
The two BC models differed in the coding of the familiarization runs. The first model relied on a simple multi-layer perceptron (MLP from multi-layer perceptron ) to independently encode state-action pairs, and the second model relied on a more complex bidirectional recurrent neural network (RNN from recurrent neural network ) to sequentially encode state pairs -action.
The states were encoded by a convolutional neural network (CNN) that was pre-trained using extended temporal contrast (ATC from augmented temporal contrast ). For both MLP and RNN encoders, the model obtained the agent's characteristic embedding by first pooling the frame-by-frame embeddings (using the mean for MLP and last step for RNN) for each familiarization trial, and then averaging the second value for the familiarization trials. When combining frames, the videos were subjected to random subsampling to use no more than 30 frames. To predict the agent's future actions, defined as a continuous position change based on video (at 3 frames per second), the models combined the feature embedding with the current state of the environment (also encoded with a CNN).
One video model sequentially encoded each familiarization trial by running up to 30 frames through a CNN and then combining them with a bidirectional RNN. The model obtained the agent's characteristic embedding by averaging the RNN embeddings. The model combined the feature embedding with the current state of the environment (defined by the current video frame) to predict the next video frame (at 3 frames per second) using the U-net architecture.
Prior to testing, the models were trained on thousands of background examples provided by the BIB dataset of BIB-like agents demonstrating simple behavior in grid space. The training sample included individual components of the test sample (for example, the movement of agents to objects, consistent goals of agents, obstacles, tools, etc. ).
In one training task, the agent moved to the same object in different places in the grid space. In the second training task, two objects were presented at different points in space, but always very close to the agent; the agent sequentially moved to one of them. In the third training task, the agent moved to one object at different points in space; at different points of acquaintance, this agent was replaced by another agent. Finally, in the fourth training task, the agent and the key were surrounded by a green barrier; the agent received a key to leave the blocked area and go to the object.
Image #4
The graphs above show the average Z-scores of unexpected model scores against expected and unexpected outcomes for each task. For comparison, the average values of the observation time of infants in the tasks from experiment No. 2 were also plotted on the graphs. It can be seen that the performance of the models bear little resemblance to the performance of infants.
To evaluate the attribution of machine goals to infants, the researchers compared the performance of models and infants on this task. Unlike infants, who paired agent and object (target) rather than agent and object position, models either associated agents with the object's position (BC MLP) or did not associate it with either the object or its position at all (BC RNN, video model).
Then the rationality attributions of the models and infants were compared in the framework of the "effective agent" and "inefficient agent" tasks. The models were successful in attributing rational actions to agents in the efficient agent task (even more so than infants). However, they did not attribute rational action to previously inefficient agents that act under new conditions in the "inefficient agent" task. Here, the results of the models were nearly orthogonal to those of the infants.
Comparison of the performance of the model and the infant in three other BIB tasks did not reveal cases where the models showed positive predictions of agent actions that were absent in the infants' predictions. In particular, while infants may have been relatively more surprised by the appearance of a new agent in the expected outcome of the multiple agent task, the models showed no difference in surprise.
In the "unreachable target" task, the video model was more surprised when the agent moved to a new object when its target object was available, unlike babies. But, given the failure of this model in the "target" and "several agents" tasks, its effectiveness is unlikely to reflect the understanding of the purposeful actions of agents in relation to objects.
For example, the model could learn that obstacles in the mesh world are blocking objects, and that agents are moving towards the objects. This would result in a lower surprise score when the agent moved to one available object compared to when it moved to any of the available objects.
Similarly, on the task of instrumental action, the models seemed to succeed where infants did not, showing more surprise when the agent moved towards the key when it was not necessary. But a closer look at the performance of the models showed that this apparent success was limited to test trials in which the green barrier was absent rather than present but not significant.
For a more detailed acquaintance with the nuances of the study, I recommend looking into the scientists' report.
Epilogue
The human brain is an amazing mechanism that is constantly working, performing many functions, many of which we are not even aware of. However, this body requires training to reach its full potential. Children in the period of early development are extremely attentive to everything that surrounds them. They still do not know how to speak and are not even able to roll over without help, but their eyes, ears, nose already read sensory information, and the brain analyzes it. In aspects of socialization, it is important to understand what a person wants. And this is not a philosophical question. This is a question of cognitive thinking, the answer to which is the correct comparison of the actions of a person and the goals that he wants to achieve with these actions. Infants, despite their very little experience of social life, have a highly developed common sense psychology, which is responsible for the connection between the agent (person) and the goal.
For artificial intelligence to truly resemble what we see in movies and read about in books, it must also have certain cognitive skills. However, as the study we reviewed today showed, in some aspects AI lags behind even 11-month-old babies.
Scientists conducted a series of tests in which, with the help of primitively animated videos, they were able to assess the degree of psychology of the common sense of babies. The video featured a gridded space, an agent, and an object (target). The agent moved across the field to the object. In some cases, there was an obstacle between the agent and the tree, which could be bypassed using a key (another object). Also, the agents could behave effectively or inefficiently, use the key when it is not needed, etc. All variations in the behavior of the agents caused a certain reaction in the infants (longer observation of the agent), which it was decided to call simply “surprise”.
The scientists then prepared several neural network models and tested them similarly. In some of them, the models seemed to perform better than the infants at first glance, but closer analysis showed that this was only the case in test runs.
Summing up the results of tests with babies and models, as well as their comparison, scientists came to the conclusion that even the most advanced AI cannot yet surpass babies in the framework of common sense psychology.
And this is especially surprising, because a lot of knowledge can be invested in a model, thousands of training tests can be carried out, but it will still cope worse than a little man who also cannot crawl.
According to the authors of the study, their work not only shows that the human brain (even an infant) is superior to AI in many ways, but also provides a direction for future research and development. When designing an AI that should mimic the human brain, it is necessary to clearly understand which aspects of the brain are important in order to achieve a full-fledged simulation.