How does chunking help your memory
How does chunking help working memory?
. 2019 Jan;45(1):37-55.
doi: 10.1037/xlm0000578. Epub 2018 Apr 26.
Mirko Thalmann 1 , Alessandra S Souza 1 , Klaus Oberauer 1
Affiliations
Affiliation
- 1 Department of Psychology, Cognitive Psychology Unit, University of Zurich.
- PMID: 29698045
- DOI: 10.1037/xlm0000578
Free article
Mirko Thalmann et al. J Exp Psychol Learn Mem Cogn. 2019 Jan.
Free article
. 2019 Jan;45(1):37-55.
doi: 10.1037/xlm0000578. Epub 2018 Apr 26.
Authors
Mirko Thalmann 1 , Alessandra S Souza 1 , Klaus Oberauer 1
Affiliation
- 1 Department of Psychology, Cognitive Psychology Unit, University of Zurich.
- PMID: 29698045
- DOI: 10.
1037/xlm0000578
Abstract
Chunking is the recoding of smaller units of information into larger, familiar units. Chunking is often assumed to help bypassing the limited capacity of working memory (WM). We investigate how chunks are used in WM tasks, addressing three questions: (a) Does chunking reduce the load on WM? Across four experiments chunking benefits were found not only for recall of the chunked but also of other not-chunked information concurrently held in WM, supporting the assumption that chunking reduces load. (b) Is the chunking benefit independent of chunk size? The chunking benefit was independent of chunk size only if the chunks were composed of unique elements, so that each chunk could be replaced by its first element (Experiment 1), but not when several chunks consisted of overlapping sets of elements, disabling this replacement strategy (Experiments 2 and 3). The chunk-size effect is not due to differences in rehearsal duration as it persisted when participants were required to perform articulatory suppression (Experiment 3). Hence, WM capacity is not limited to a fixed number of chunks regardless of their size. (c) Does the chunking benefit depend on the serial position of the chunk? Chunks in early list positions improved recall of other, not-chunked material, but chunks at the end of the list did not. We conclude that a chunk reduces the load on WM via retrieval of a compact chunk representation from long-term memory that replaces the representations of individual elements of the chunk. This frees up capacity for subsequently encoded material. (PsycINFO Database Record (c) 2018 APA, all rights reserved).
Similar articles
-
Chunking and redintegration in verbal short-term memory.
Norris D, Kalm K, Hall J. Norris D, et al. J Exp Psychol Learn Mem Cogn. 2020 May;46(5):872-893. doi: 10.1037/xlm0000762. Epub 2019 Sep 30. J Exp Psychol Learn Mem Cogn. 2020. PMID: 31566390 Free PMC article.
-
Infants hierarchically organize memory representations.
Rosenberg RD, Feigenson L. Rosenberg RD, et al. Dev Sci. 2013 Jul;16(4):610-21. doi: 10.1111/desc.12055. Epub 2013 Apr 10. Dev Sci. 2013. PMID: 23786478
-
Topology and graph theory applied to cortical anatomy may help explain working memory capacity for three or four simultaneous items.
Glassman RB. Glassman RB. Brain Res Bull. 2003 Apr 15;60(1-2):25-42. doi: 10.1016/s0361-9230(03)00030-3. Brain Res Bull. 2003. PMID: 12725890 Review.
-
Seven-month-old infants chunk items in memory.
Moher M, Tuerk AS, Feigenson L. Moher M, et al.
J Exp Child Psychol. 2012 Aug;112(4):361-77. doi: 10.1016/j.jecp.2012.03.007. Epub 2012 May 9. J Exp Child Psychol. 2012. PMID: 22575845 Free PMC article.
-
Chunking and data compression in verbal short-term memory.
Norris D, Kalm K. Norris D, et al. Cognition. 2021 Mar;208:104534. doi: 10.1016/j.cognition.2020.104534. Epub 2020 Dec 21. Cognition. 2021. PMID: 33360054 Review.
See all similar articles
Cited by
-
Chunking, boosting, or offloading? Using serial position to investigate long-term memory's enhancement of verbal working memory performance.
Bartsch LM, Shepherdson P. Bartsch LM, et al. Atten Percept Psychophys. 2022 Dec 1. doi: 10.3758/s13414-022-02625-w.
Online ahead of print. Atten Percept Psychophys. 2022. PMID: 36456795
-
Facilitative Effects of Embodied English Instruction in Chinese Children.
Guan CQ, Meng W. Guan CQ, et al. Front Psychol. 2022 Jul 14;13:915952. doi: 10.3389/fpsyg.2022.915952. eCollection 2022. Front Psychol. 2022. PMID: 35911001 Free PMC article.
-
Towards Digestible Digital Health Solutions: Application of a Health Literacy Inclusive Development Framework for Peripartum Depression Management.
Zingg A, Singh T, Myneni S. Zingg A, et al. AMIA Annu Symp Proc. 2022 Feb 21;2021:1274-1283. eCollection 2021. AMIA Annu Symp Proc. 2022. PMID: 35308913 Free PMC article.
-
Twelve quick tips for software design.
Wilson G. Wilson G. PLoS Comput Biol. 2022 Feb 24;18(2):e1009809. doi: 10.1371/journal.pcbi.1009809. eCollection 2022 Feb. PLoS Comput Biol. 2022. PMID: 35202401 Free PMC article. No abstract available.
-
The Time-Course of the Last-Presented Benefit in Working Memory: Shifts in the Content of the Focus of Attention.
Valentini B, Uittenhove K, Vergauwe E. Valentini B, et al. J Cogn. 2022 Jan 7;5(1):8. doi: 10.5334/joc.199. eCollection 2021. J Cogn. 2022. PMID: 35083411 Free PMC article.
See all "Cited by" articles
MeSH terms
Grant support
- Swiss National Science Foundation
What Is Chunking & How To Use This Powerful Memory Strategy
For most of us, our memories have their limits!
Chunking helps you overcome the natural limitations of your memory, and is therefore a very powerful trick for helping you to learn information and get it into memory.
Read on to discover what chunking is, and to explore the evidence for just how much chunking can improve your capacity to remember things.
I’ll share a wealth of handy “trade secrets” for applying this theory to your studies, a treasure-trove of techniques you can use to make memorisation far easier, using learning by chunking.
Some you may be familiar with.
Some will almost certainly be new.
All will make your life far easier if you’ve got a lot of information to learn for tests and exams.
Let’s do this:
What is chunking
So what exactly is “chunking”?
Come to that, what is a “chunk”?
We need to go back to George Miller and his “Magic Number Seven”.
Miller’s “Magic Seven”
“I have been persecuted by an integer [a whole number]”, George Miller wrote, “for seven years this number has followed me round”.
George was a psychologist, and he was referring to the number “seven” which seemed to come up remarkably often as about the limit of the number of discrete pieces of information we can remember at any one time.
The information might be words, numbers, locations: it didn’t really matter – most people can remember at least 5-6 “units of information”, and no more than 8-9 “units”, with seven being the most commonly-observed number people could handle.
So much so, it’s often known as “Miller’s Magic Number Seven (plus or minus two)”.
If “seven” is the limitation, then chunking is the hack to get round it.
Chunking definition and demonstration
The APA Dictionary of Psychology defines “chunking” as “the process by which the mind divides large pieces of information into smaller units (chunks) that are easier to retain in short-term memory… one item in memory can stand for multiple other items”.
Each individual “chunk” is a group of information units – words, numbers, phrases – that are strongly related to each another, but fairly unrelated to information in other groups (ref.) So “chunking” describes the process of grouping related bits of information together, effectively reducing the number of “things” you need to remember.
If you’re technologically minded, you can think of chunking as being a little like a compression algorithm in your computer or smartphone that allows large image or video files to take up less space on your device’s memory.
This is best understood with an example.
Give yourself five seconds to look at the letters below, then look away and try and remember as many of the 18 letters as you can.
What is chunking – try and remember these lettersNot easy.
Let’s try again:
Below are the same letters, but with the order reversed, and grouped to make meaningful 3-letter strings.
You could take it a step further if you want, and categorise into three groups, by theme.
What is chunking – much easier to remember 3 categories than 18 lettersSo rather than 18 separate pieces of information – 18 letters – you’ve reduced it down to just 3 concepts: animals, companies, alphabet. That’s much easier to remember!
The power of chunking
Scientists have demonstrated how chunking helps facilitate faster learning and easier memorisation in a wide range of situations:
- Learning locations or positions
- Reading and learning music
- Learning words and letters
- Memorising strings of numbers – in this dramatic example, a subject of average intelligence used a chunking strategy based largely on running times to remember strings of up to eighty digits – over 10x Miller’s Magic Seven!
There is also good evidence that our sensory systems automatically “chunk” incoming sounds and sights to help you process the world faster and more easily, without you giving it a second thought.
Chess players seem to “chunk” the positions of pieces on the board into common patterns, allowing them to process what’s happening on the board more easily than if they had to process the position of each piece individually.
You’ve probably been using chunking for years without realising it, in order to remember your phone number.
I have the phone number for my childhood home etched into my memory as “09982-330-508”, grouping those 11 digits into groups of five, three and three. (I’ve changed a few of the numbers to protect the privacy of whoever lives in that house now).
But if you gave me that same set of numbers with different grouping – e.g. “09-9823-305-08” – I’d have real problems recognising whether that really was my number or not!
How do you group the digits in your phone number?
Try grouping the digits differently, speak it out loud and see how quickly your familiar number can start to feel alien. That’s the power of chunking.
Chunking memory techniques
So chunking more than just an abstract psychological principle, it has powerful practical applications, particularly for students trying to get information into memory for tests and exams.
In the examples below, I’ll share some “trade secrets” for simplifying complex information to learn it far more easily.
These techniques are united by using grouping and patterns to reduce the number of separate items you need to commit to memory, which is what chunking is all about.
1. First letters (“acronyms”)
A popular way to use chunking for improved memory is by taking the first letters of a set of words you want to learn, and making another word from those letters: an “acronym”.
If you’ve ever had to learn the Great Lakes of North America, you may have taken the first letters of each lake –
- Huron
- Ontario
- Michigan
- Erie
- Superior
– and spelt the word “HOMES”.
This is a form of chunking because you’ve simplified five separate items down to just one: though you still need to make sure you can remember what each letter stands for.
Here’s another example of this in action, with some slightly more complex information that will be familiar to students of business or finance:
Chunking memory techniques: acronymsAn acronym is more easily remembered if it’s a real word (like “homes”), but can still be helpful if it’s a pronounceable nonsense word (e.g. “R GON VFIT”). You can always add an extra letter or two in your head to improve pronounceability and perhaps incorporate a little meaning – e.g. “aRe GOiN’ V. FIT”, sounds a little like you’re embarking on a rigorous new exercise regime.
2. Made up phrases (“acrostics”)
The second technique uses phrases: “acrostics”.
An “acrostic” is a bit like an acronym in that it takes the first letters of the words you’re trying to remember, but rather than make a single word from the first letters, you assign each letter a new word to make a memorable phrase.
For the Great Lakes, rather than “homes” (an acronym), you could make a phrase from the first letters, like “Hovering On My Extreme Surfboard”.
This particular acrostic has the advantages of being slightly water-related, in keeping with the “lakes” theme, and also suggests a crazy, and therefore memorable, image of a floating surfboard.
For instance, you may have come across the acrostic “Please Excuse My Dear Aunt Sally”, for remembering the order of operations in math(s): Parentheses, Exponents, Multiplication, Division, Addition, Subtraction.
For a crazier mental image, try “Please Email My Dad A Shark” (credit to Randall Munroe for that one).
If you’re trying to come up with an acrostic yourself, there are free tools available to help, like this one.
Given the choice, I’d generally go for an acronym over an acrostic – they’re much easier to “decode”, requiring only two steps rather than three:
Chunking memory techniques: acronyms vs acrostics3. Chunking lists using associated concepts
The third strategy is all about making lists easier to remember. Any time I’ve got a list that’s got more than about five items in it, I look for opportunities to simplify through chunking.
If you’re going shopping, much easier to remember two things (“pancakes and burritos”) than twelve (flour, eggs, milk, syrup, lemon, tortillas, chicken breast, rice, peppers, avocado, lettuce, rice). Sure, you need to know the ingredients for each recipe: you might already know that, but even if you don’t, chances are that adding a little structure to your shopping list by grouping related items together will make it much easier to remember.
You can look for all kinds of associations, depending on your level of prior knowledge, and how much meaning items on the list have for you.
See the figure below for some inspiration. Hopefully there’s a way of grouping which adds meaning (as in examples #1 and #2 below), but if you really have to, you can always fall back on how linguistic similarities (example #3 below).
Chunking memory tricks: examples of associated meanings for learning EU countriesThis whole example is full of mini-examples of how to do learning by chunking effectively. Say you’ve decided to go for example #1, grouping by geography. You’ll need to make sure you can remember what your category headings are, and the number of items under each category heading.
Chunking can help us out on both counts:
- For the different categories, how about an acrostic: “Never Eat Shredded Wheat Cereal” for “Northern Eastern Southern Western Central”.
- For the number of items in each category, maybe you can remember the string of numbers in order: “33443344”. Make it easier by adding some grouping, “phone number” style, e.g. “33-44-33-444”. Much better.
Same idea for the language groups example: the acronym of the first letters makes a pronounceable word “AHN-BIGS” – and the numbers make an ascending sequence 1234567.
4. Chunking to learn related numbers
One final trick if you have a lot of numbers to remember.
This works a treat for dates in history, for example, provided you’re reasonably comfortable with basic arithmetic.
If you’ve got a long list of dates to learn, the first step, as before, is to group them by related concepts. Maybe 1776, 1781 and 1788 are the key dates of battles in a notable war: learn those as a group.
Instead of learning each date individually, you might find it easier to remember how they are related. In the example below, rather than learning 12 digits’ worth of information, you can reduce the work you need to do by just remembering the first date (1776 – 4 digits) and two further digits (5 and 7) for the year intervals between the dates.
Learning by chunking: numbers and datesLook for patterns to remember strings of dates more easily
To give you some other ideas for how this can work in practice, the two dates often stated for the fall of the Roman Empire are 476, when Ancient Rome (in Italy) and the Western Roman Empire was defeated by the Goths, and 1453, when Constantinople and the Roman Empire in the East fell to the Ottoman Empire.
It probably doesn’t help much to learn the difference between the two dates (977 in this case). Better, perhaps to look for other patterns.
- These dates are nicely separated by approximately 1,000 years – so we know the first digits for each date should be “4” and “14”, respectively.
- You could also remember that the final digit for 1453 is half the value of the final digit in 476.
- Which just leaves the “5” in 1453 and the “7” in 476 to remember – perhaps remembering them as a pair is useful, with a difference of two between them.
You can even use patterns to remember a single number
Let’s go back to 1453 to demonstrate this in action:
- It may help to notice that the first three digits are a sum: 1+4 = 5. So if you can remember it’s in the 1400s, you’ll easily be able to get the “5” by adding the “1” and the “4”.
- Maybe you can remember the “3” from the 1 and 4 too: 4 minus 1 = 3.
The human brain is brilliant at pattern-finding.
Use this to your advantage to turn bland strings of digits into something meaningful by looking for the links and associations between them, cutting down the number of separate digits you need to recall individually.
Learning by chunking
So how to incorporate chunking into your studying?
On its own, chunking isn’t really a stand-alone study technique – in other words, you can’t go home tonight and get an hour’s quality chunking done!
See it more as a useful addition to your toolkit of “how to learn” strategies, and deploy it whenever you see opportunities to simplify complex information, to make it easier to remember.
One word of caution, though: watch out for times when your chunking strategy “gets in the way” of learning.
If the chunking you’re using is particularly complex or elaborate, you might find you’re making life harder for yourself, it might actually be easier to strip it away and just learn the underlying information.
For example, I mentioned a strategy to “chunk” related dates: some people (me included) find it relatively straightforward to see patterns in numbers.
But if you’re very under-confident in math(s) and find adding and subtracting a real struggle, you might prefer just learn the dates separately rather than worrying about how they are mathematically related.
Retrieval practice and spacing
Whether you’re using chunking or not, any good study routine should be grounded in retrieval practice – an extremely powerful technique for studying by practising remembering information – while spacing out your work by revisiting a topic on several different days, rather than just cramming all your time on that topic on a single day.
Chunking works very nicely with retrieval practice and spaced learning: once you’ve decided how you’re going to chunk the information, practise remembering that information using your chunking strategy (retrieval practice) on several different days (spaced learning) separated by time intervals.
You’ll often feel like you’re remembering in two stages.
- Step 1 is remembering the “big picture”: going back to the “countries of the European Union” example, that would be remembering the categories (e.g. “AHN-BIGS” in our language groups example) and the numbers in each category (1234567).
- Step 2 is remembering the details: the individual countries under each heading.
Be sure to practise both steps, and diagnose which parts of the recall process you find hardest.
Perhaps you have problems with Step 1 (remembering the categories themselves) or maybe you struggle more with Step 2, such as having issues remembering all 7 “Slavic” countries. Focus your efforts on the areas you struggle most with, and look for opportunities to group and simplify further if necessary.
You might find it’s tough to remember the information accurately the first time you leave a time interval of a few days before attempting retrieval practice – even if you’re using chunking.
Don’t worry: that’s simply because of the nature of the “forgetting curve”, and it’s why spaced learning is such a good idea. Take a moment to refresh your memory of the information, and try and remember it again. Repeat until it starts to stick, and you can reliably remember it after you haven’t looked at it for a few days.
Have fun with your chunking strategies and get creative: good luck!
Get more from the science of learning with my free cheat sheet:
- About
- Latest Posts
William Wadsworth
Founder and Director of Learning Science at Exam Study Expert
William Wadsworth is a Cambridge University educated psychologist and learning science researcher. He got top 0.01% exam results in the UK as a student over 10 years ago, and ever since has been obsessed with helping subsequent generations of students ace their exams, through the science of studying smarter, not harder. Half a million students in 150+ countries follow his advice through this site and the Exam Study Expert podcast, and he’s the best-selling author of the “ingenious” guide to test-taking strategy, Outsmart Your Exams. To get in touch with William, including to find out more about his transformational 1:1 coaching sessions, please click here.
Latest posts by William Wadsworth (see all)
Combating Memory Fragmentation in the Linux Kernel / Sudo Null IT News
This two-part compilation provides common methods for preventing memory fragmentation in Linux, as well as understanding how to compact memory, how to view the fragmentation index, and more.
(External) memory fragmentation has been a longstanding problem in Linux kernel programming. During operation, the system assigns various tasks to memory pages, which gradually become fragmented as a result, and eventually a busy system that is in operation for a long time may have only a few contiguous physical pages.
Because the kernel supports virtual memory management, physical fragmentation is often not a problem. When using page tables, unless the pages are too large, the physically scattered memory in the virtual address space is still contiguous.
However, it becomes very difficult to allocate contiguous memory from the kernel's linear mapping area. For example, it is difficult to allocate structural objects via an allocator - a typical and frequent operation in kernel mode - or to work with a direct memory access (DMA) buffer that does not support modes scatter/gather
. Such operations can cause frequent memory compaction, resulting in fluctuations in system performance or allocation failure. In the process of slow (slow path) memory allocation, various operations are performed, determined by a flag on the allocation page.
If kernel programming is no longer based on the allocation of top-level physical memory in a linear address space, then the problem of fragmentation will be solved. However, for a project as large as the Linux kernel, making such changes would be impractical.
Since Linux 2.x, the community has tried many ways to deal with the fragmentation problem, including many effective, albeit unusual, patches. Some of the improvements made were controversial, such as the memory compaction mechanism. At the LSFMM 2014 conference, many developers complained about the low efficiency of this mechanism and the difficulty of reproducing errors. But the community did not abandon this functionality and continued to optimize it in subsequent versions.
Mel Gorman was the most diligent participant in this project. He brought two sets of important patches to it. The first one was included in Linux 2.6.24 and went through 28 versions before it was accepted by the community. The second set was added already in Linux 5.0 and successfully reduced fragmentation by 94% for machines with one or two sockets.
In this article, I'll introduce a number of common extensions to the buddy memory allocation algorithm that helps prevent memory fragmentation in the Linux 3.10 kernel, explain the principles of memory compaction, learn how to view the fragmentation index, and quantify the unnecessary latency caused by memory compaction.
▍A brief history of defragmentation
Before I begin, I would like to recommend a good read to you. The following articles describe all the efforts that have gone into optimizing high-level memory allocation in the Linux kernel.
Publication date | Articles on LWN.net |
2004-09-08 | Kswapd and high-order allocations |
2004-05-10 | Active memory defragmentation |
2005-02-01 | Yet another approach to memory fragmentation |
2005-11-02 | Fragmentation avoidance |
2005-11-08 | More on fragmentation avoidance |
2006-11-28 | Avoiding - and fixing - memory fragmentation |
2010-01-06 | Memory compaction |
2014-03-26 | Memory compaction issues |
2015-07-14 | Making kernel pages movable |
2016-04-23 | CMA and compaction |
2016-05-10 | Make direct compaction more deterministic |
2017-03-21 | Proactive compaction |
2018-10-31 | Fragmentation avoidance improvements |
2020-04-21 | Proactive compaction for the kernel |
Well, now you can start.
▍Twin Algorithm
Linux uses a simple and efficient twin algorithm as its page allocator. At the same time, some improvements were made to its classic version:
- allocation of memory sections;
- allocation of page sets for each individual CPU;
- grouping by migration types.
The Linux kernel uses the terms node, zone, and page to describe physical memory. The partition allocator focuses on a specific zone in a specific node.
Prior to version 4.8, the kernel implemented zone-based page processing because the early design was oriented primarily towards 32-bit processors and had a lot of top-level memory. However, the rate of page aging in different zones of the same site turned out to be inconsistent, which caused many problems.
Over a fairly long period of time, the community has added a lot of all sorts of patches, but the problem remains. In light of the increasing use of 64-bit processors and large amounts of memory, Mel Groman moved the strategy of returning pages from the zone to the node, which solved the problem. If you are using Berkley Packet Filter (BPF) tools to monitor return operations, then you would like to know this.
The per-CPU page set allocation method optimizes single page allocation, thereby reducing the frequency of processor mutex conflicts. It has nothing to do with defragmentation.
Grouping by move type is a defragmentation method that I will discuss in detail.
▍Group by movement type
First you need to understand the layout of the memory address space. Each processor architecture has its own definition. For example, the definition for x86_64 is in mm.txt.
Since virtual and physical addresses do not map linearly, accessing virtual space through the page table (eg, requiring dynamic memory allocation in user space) does not require physical memory continuity. Take Intel's five-level page table as an example, where the virtual address is broken down from bottom to top:
- Bottom level: page offset;
- Level 1: immediate page index;
- Level 2: middle directory index;
- Level 3: top directory index;
- Level 4: Level 4 directory index;
- Level 5: Global page index.
Five-level paging on Intel systems
The physical memory page frame number is stored in the first level page table entry and can be found by the corresponding index. The physical address is a combination of the found frame number and the page offset.
Imagine that you want to change the corresponding physical page in a first-level table entry. To do this, it will be enough:
- Select a new page.
- Copy data from the old page to the new one.
- Change the value of the first level page table entry to the new page frame number.
These operations will not change the original virtual address, and you can migrate such pages as you wish.
In the linear mapping area, a virtual address is represented as a physical address plus a constant. Changing the physical address leads to a change in the virtual one, as a result of which access to the latter causes an error. Therefore, these pages are not recommended to be migrated.
When physical pages accessed through a page table and pages accessed through a linear map are mixed and managed together, memory fragmentation occurs. As a result, several types of memory relocation are defined in the kernel, and pages for defragmentation are grouped by these types.
The three most commonly used memory move types are: MIGRATE_UNMOVABLE
, MIGRATE_MOVABLE
and MIGRATE_RECLAIMABLE
. Other types have a special purpose, which I will not discuss here.
The distribution of each move type at each stage can be viewed via /proc/pagetypeinfo
:
The allocation flag applied to a page determines which move group it is allocated from. For example, for userspace memory, you can use
__GFP_MOVABLE
, and for pages __GFP_RECLAIMABLE
.
When pages of a particular type are exhausted, the kernel removes physical pages from other groups. To avoid fragmentation, this extraction starts with the largest block of pages. The block size is defined in pageblock_order
.
The standby priorities of the three move types listed, in order from top to bottom, are:
MIGRATE_UNMOVABLE: MIGRATE_RECLAIMABLE, MIGRATE_MOVABLE0212The kernel introduces grouping by migration type for defragmentation purposes. However, frequent page loss indicates the presence of external memory fragmentation events, and these may cause problems in the future.
▍External memory fragmentation event analysis
In my previous article Why We Disable Linux's THP Feature for Databases, I mentioned that you can use the
ftrace
events provided by the kernel to analyze external memory fragmentation.The procedure in this case is as follows:
1. Enable events
ftrace
:echo 1> /sys/kernel/debug/tracing/events/kmem/mm_page_alloc_extfrag/enable2. Start collecting events
ftrace
:9021 catkernel debug/tracing/trace_pipe> ~/extfrag.log
3. Stop collecting by pressing Ctrl+C. The event contains many fields:
To analyze the number of external memory fragmentation events, focus on those containing fallback_order < pageblock order
. On x86_64 , pageblock order
is 9.
4. Clear event:
echo 0> /sys/kernel/debug/tracing/events/kmem/mm_page_alloc_extfrag/enable
Here we see that grouping by movement types only delays fragmentation, but does not solve the problem at the root.
Increased fragmentation and lack of contiguous physical memory affect performance. This means that the described measure alone is not enough.
Memory seal
Prior to the implementation of the principle of memory compaction in the kernel, lumpy reclaim was used for defragmentation. However, in version 3.10 (currently the most common), this functionality was removed. If you are interested in learning more about this technique, you can read the materials that I cited in the article A brief history of defragmentation. Here I will immediately move on to the topic of memory compaction.
▍Introduction of the algorithm
The Memory Compaction article on LWN.net details the algorithmic principle of memory compaction. A simple example is the following fragmented zone:
Small fragmented memory zone - LWN.net
The white blocks are free pages and the red blocks are dedicated pages. The compaction of memory in relation to this zone is divided into three main stages:
1. Scanning the zone from left to right in search of red pages with type MIGRATE_MOVABLE
.
Search for moving pages
2. At the same time, scan the area from right to left to search for free pages.
Search for free pages
3. Shift the moved pages under the free ones to create a continuous section of free space.
Compacted memory zone
The principle itself looks relatively simple, and the kernel also provides /proc/sys/vm/compact_memory
to start memory compaction manually.
However, as mentioned at the beginning of this article, memory compaction in practice turns out to be particularly effective - at least not for the most common v3.10 - regardless of manual or automatic start. In view of the associated computational load, this operation, on the contrary, leads to the formation of a bottleneck.
Although the community members did not abandon this idea and continued to optimize it. For example, the kcompactd tool was later added to the v4.6 kernel, and direct compaction was made more specific in v4. 8.
▍When memory compaction is performed
In the v3.10 kernel, this operation is performed in any of the following situations:
- Call thread
kswapd
to balance zones after a failed top-level allocation. - Thread call
khugepaged
to merge small memory chunks into large pages. - Activate memory compaction manually via interface
/proc
.
The system resorts to immediate memory reclaiming, including the handling of Transparent Huge Pages (THP) failure exceptions, to meet the requirement to allocate higher-level memory.
THP functionality slows down performance, so it is recommended to disable this option. I will not analyze this nuance here and will focus mainly on the process of memory allocation.
Slow path memory allocation
If no available pages are found in the allocator lists during allocation, the following occurs:
- limit.
- If the allocation fails, indicating a slight shortage of memory, the allocator wakes up the
kswapd
thread to return the pages asynchronously and retry the allocation, also using the lower limit as the threshold. - Failure of this operation will mean a serious lack of memory. In such a case, the kernel first runs asynchronous memory compaction.
- If this allocation also fails after the asynchronous compaction, the kernel reclaims the memory directly.
- If the kernel then returns not enough free pages to meet the requirements, it performs direct memory compaction. If no pages could be freed, the OOM Killer is called to return the memory.
The steps listed are only a simplified description of the actual flow. In reality, it is more complicated and differs depending on the level of requested memory and allocation flags.
As for the direct return, it is performed only in case of a serious lack of memory, and also due to its fragmentation in practical scenarios. At certain times, both of these situations can occur simultaneously.
▍Memory compaction analysis
Latency quantification
As discussed in the previous section, the kernel can reclaim or compact memory when allocating memory. To make it easier to quantify the latency caused by direct memory reclaiming and compacting, I added two tools to the BCC project, drsnoop and compactsnoop.
They are both based on kernel events and well documented, but I do want to clarify one thing: to reduce the cost of introducing Berkeley Packet Filters (BPFs), these tools intercept the delay of each respective event. Therefore, it can be seen from the output that each memory request has multiple latency results.
The reason for this one-to-many relationship is that for older versions of the kernel like v3.10 it is not clear how many times the kernel will try to reclaim memory over the slow path. This uncertainty also causes OOM Killer to start running too early or too late. As a result, most tasks on the server are suspended for a long time.
After the implementation of the patch mm: fixed 100% CPU kswapd busyloop on unreclaimable nodes in v4.12, the maximum number of direct memory reclaim operations was limited to 16. Let's assume that the average delay of such an operation is 10 ms. (Pruning active or inactive LRU chain tables is costly on modern servers with several hundred gigabytes of RAM. Additional latency is also imposed if the server has to wait for a dirty page writeback.)
If a thread asks for a page allocator and after one immediate return receives empty memory, the return delay increases to 10 ms. If the kernel had to perform 16 such operations to return enough memory, the total latency for the entire allocation process would be already 160 ms, causing a serious performance hit.
View fragmentation index
Let's get back to compacting memory. The basic logic of this process is divided into four steps:
- Determine if a memory area is suitable for compaction.
- Sets the frame number of the start page to be scanned.
- Isolate pages of type
MIGRATE_MOVABLE
. - Move pages of type
MIGRATE_MOVABLE
to the top of the zone.
If, after one move, the zone still needs compaction, the core repeats this cycle three to four times until compaction is complete. This operation consumes a lot of processor resources, and therefore, when monitoring, you can often see its full load.
Okay, so how does the kernel determine if a zone is suitable for compacting memory?
If you use interface /proc/sys/vm/compact_memory
to force compaction of a zone's memory, then the kernel does not need to determine its suitability for this procedure.
If compaction is enabled automatically, the kernel calculates a fragmentation index of the requested level, determining whether there is enough memory left in the zone for compaction.
The closer the resulting index is to 0, the higher the probability of this operation failing due to lack of memory. This means that in such a case, a memory return operation is more suitable. An index approaching 1,000 increases the likelihood of an allocation failure due to excessive external fragmentation. Therefore, memory reclaiming rather than compaction should also be used in this situation.
The choice of the kernel in favor of compacting or returning memory is determined by the external fragmentation threshold, which can be viewed through the interface /proc/sys/vm/extfrag_threshold
.
You can also view the fragmentation index directly with cat /sys/kernel/debug/extfrag/extfrag_index
. Please note that the results below are divided by 1,000:
Pros and cons of
Memory compaction analysis can be done both by monitoring interfaces based on the file system /proc
, or with kernel event-based tools ( drsnoop
and compactsnoop
), but each has its own strengths and weaknesses.
Interface monitoring is easy to use, but it will not quantify latency results and the sampling period will be very long. Kernel-based tools, in turn, solve these problems, but in this case, you need to have a solid understanding of how the kernel subsystems work, and besides, there are certain requirements for its client version.
In fact, these methods complement each other well. Using both of them, you can fully analyze memory compaction.
Anti-fragmentation
The kernel has special mechanisms for working with slow backend devices. For example, it implements a second chance page replacement method, as well as a preset range based on the LRU algorithm, and there is no way to limit the allocation of part of the memory for the page cache ( page cache
). Some companies have customized the kernel to fit their needs to limit the page cache, and have even offered to implement these versions to the community, but they have been rejected. I think the reason is that this functionality causes a number of problems like preset working settings.
In this regard, in order to reduce the frequency of memory return operations and in order to combat fragmentation, it would be a good decision to increase vm.min_free_kbytes
(up to 5% of total memory). This will indirectly limit the share of the page cache in scenarios with a large number of I / O operations and in cases where the machine has more than 100GB of memory installed.
Although increasing vm.min_free_kbytes
results in some memory overhead, the overhead is negligible. For example, if the server's storage is 256GB and you set vm.min_free_kbytes
to 4G, that would only be 1.5% of the total space.
The community, of course, also noticed this memory cost, so a patch was added in v4.6 to optimize it accordingly.
Alternatively, can be done at the right time drop cache
, but this can lead to fluctuations in application performance.
Conclusion
At the beginning of the article, I briefly explained why external fragmentation affects performance and talked about the efforts made by the community in terms of defragmentation, after which I talked about the basic principles of defragmentation used in the v3.10 kernel, as well as ways to qualitatively and quantitatively evaluate fragmentation.
I hope you found this material helpful. If you have any thoughts on the topic of Linux memory management, feel free to discuss them in our TiDB Community workspace on Slack.
Approx. Lane: The original articles by Wenbo Zhang are available here (Part 1) and here (Part 2).
Fighting memory fragmentation in the Linux kernel
This two-part compilation provides common methods for preventing memory fragmentation in Linux, as well as understanding how to compact memory, how to view the fragmentation index, and more.
(External) memory fragmentation has been a longstanding problem in Linux kernel programming. During operation, the system assigns various tasks to memory pages, which gradually become fragmented as a result, and eventually a busy system that is in operation for a long time may have only a few contiguous physical pages.
Because the kernel supports virtual memory management, physical fragmentation is often not a problem. When using page tables, unless the pages are too large, the physically scattered memory in the virtual address space is still contiguous.
However, it becomes very difficult to allocate contiguous memory from the kernel's linear mapping area. For example, it is difficult to allocate structural objects via an allocator - a typical and frequent operation in kernel mode - or to work with a direct memory access (DMA) buffer that does not support modes scatter/gather
. Such operations can cause frequent memory compaction, resulting in fluctuations in system performance or allocation failure. In the process of slow (slow path) memory allocation, various operations are performed, determined by a flag on the allocation page.
If kernel programming is no longer based on the allocation of top-level physical memory in a linear address space, then the problem of fragmentation will be solved. However, for a project as large as the Linux kernel, making such changes would be impractical.
Since Linux 2.x, the community has tried many ways to deal with the fragmentation problem, including many effective, albeit unusual, patches. Some of the improvements made were controversial, such as the memory compaction mechanism. At the LSFMM 2014 conference, many developers complained about the low efficiency of this mechanism and the difficulty of reproducing errors. But the community did not abandon this functionality and continued to optimize it in subsequent versions.
Mel Gorman was the most diligent participant in this project. He brought two sets of important patches to it. The first one was included in Linux 2.6.24 and went through 28 versions before it was accepted by the community. The second set was added already in Linux 5.0 and successfully reduced fragmentation by 94% for machines with one or two sockets.
In this article, I'll introduce a number of common extensions to the buddy memory allocation algorithm that helps prevent memory fragmentation in the Linux 3.10 kernel, explain the principles of memory compaction, learn how to view the fragmentation index, and quantify the unnecessary latency caused by memory compaction.
▍A brief history of defragmentation
Before I begin, I would like to recommend a good read to you. The following articles describe all the efforts that have gone into optimizing high-level memory allocation in the Linux kernel.
Publication date | Articles on LWN.net |
2004-09-08 | Kswapd and high-order allocations |
2004-05-10 | Active memory defragmentation |
2005-02-01 | Yet another approach to memory fragmentation |
2005-11-02 | Fragmentation avoidance |
2005-11-08 | More on fragmentation avoidance |
2006-11-28 | Avoiding - and fixing - memory fragmentation |
2010-01-06 | Memory compaction |
2014-03-26 | Memory compaction issues |
2015-07-14 | Making kernel pages movable |
2016-04-23 | CMA and compaction |
2016-05-10 | Make direct compaction more deterministic |
2017-03-21 | Proactive compaction |
2018-10-31 | Fragmentation avoidance improvements |
2020-04-21 | Proactive compaction for the kernel |
Well, now you can start.
▍Twin Algorithm
Linux uses a simple and efficient twin algorithm as its page allocator. At the same time, some improvements were made to its classic version:
- allocation of memory sections;
- allocation of page sets for each individual CPU;
- grouping by migration types.
The Linux kernel uses the terms node, zone, and page to describe physical memory. The partition allocator focuses on a specific zone in a specific node.
Prior to version 4.8, the kernel implemented zone-based page processing because the early design was oriented primarily towards 32-bit processors and had a lot of top-level memory. However, the rate of page aging in different zones of the same site turned out to be inconsistent, which caused many problems.
Over a fairly long period of time, the community has added a lot of all sorts of patches, but the problem remains. In light of the increasing use of 64-bit processors and large amounts of memory, Mel Groman moved the page recycling strategy from the zone to the node, which solved the problem. If you are using Berkley Packet Filter (BPF) authoring tools to monitor turnover transactions, then you would like to know this.
The per-CPU page set allocation method optimizes single page allocation, thereby reducing the frequency of processor mutex conflicts. It has nothing to do with defragmentation.
Grouping by move type is a defragmentation method that I will discuss in detail.
▍Group by movement type
First you need to understand the layout of the memory address space. Each processor architecture has its own definition. For example, the definition for x86_64 is in mm.txt.
Since virtual and physical addresses do not map linearly, accessing virtual space through the page table (for example, requiring dynamic memory allocation in user space) does not require contiguous physical memory. Let's take Intel's five-level page table as an example, where the virtual address is broken down from bottom to top:
- Lower level: page offset;
- Level 1: immediate page index;
- Level 2: Medium catalog index;
- Level 3: top directory index;
- Level 4: Level 4 directory index;
- Level 5: Global page index.
Five-level paging on Intel systems
The physical memory page frame number is stored in the first level page table entry and can be found by the corresponding index. The physical address is a combination of the found frame number and the page offset.
Imagine that you want to change the corresponding physical page in a first-level table entry. To do this, it will be enough:
- Select a new page.
- Copy data from the old page to the new one.
- Change the value of the first level page table entry to the new page frame number.
These operations will not change the original virtual address, and you can migrate such pages as you wish.
In the linear mapping area, a virtual address is represented as a physical address plus a constant. Changing the physical address leads to a change in the virtual one, as a result of which access to the latter causes an error. Therefore, these pages are not recommended to be migrated.
When physical pages accessed through the page table and pages accessed through the inline map are mixed and managed together, memory fragmentation occurs. As a result, several types of memory relocation are defined in the kernel, and pages for defragmentation are grouped by these types.
The three most used types of memory move are: MIGRATE_UNMOVABLE
, MIGRATE_MOVABLE
and MIGRATE_RECLAIMABLE
. Other types have a special purpose, which I will not discuss here.
The distribution of each move type at each stage can be viewed via /proc/pagetypeinfo
:
The allocation flag applied to a page determines which move group it is allocated from. For example, for user space memory, you could use __GFP_MOVABLE
, and for pages, __GFP_RECLAIMABLE
.
When pages of a certain type are exhausted, the kernel removes physical pages from other groups. To avoid fragmentation, this extraction starts with the largest block of pages. The block size is defined in
pageblock_order
.
The standby priorities of the three move types listed, in order from top to bottom, are:
MIGRATE_UNMOVABLE: MIGRATE_RECLAIMABLE, MIGRATE_MOVABLE0212The kernel introduces grouping by migration type for defragmentation purposes. However, frequent page loss indicates the presence of external memory fragmentation events, and these may cause problems in the future.
▍External memory fragmentation event analysis
In my previous article Why We Disable Linux's THP Feature for Databases, I mentioned that you can use the
ftrace
events provided by the kernel to analyze external memory fragmentation. The procedure in this case is as follows:1. Enable events
ftrace
:echo 1> /sys/kernel/debug/tracing/events/kmem/mm_page_alloc_extfrag/enable2. Start collecting events
ftrace
:cat /sys/kernel/debug/tracing/trace_pipe> ~/extfrag.log
3. Stop collecting by pressing Ctrl+C. The event contains many fields:
To analyze the number of external memory fragmentation events, focus on those containing
fallback_order < pageblock order
. On x86_64, pageblock order
is 9.4. Clear event:
echo 0> /sys/kernel/debug/tracing/events/kmem/mm_page_alloc_extfrag/enableHere we see that grouping by movement types only delays fragmentation, but does not solve the problem at the root.
Increased fragmentation and lack of contiguous physical memory affect performance. This means that the described measure alone is not enough.
Memory seal
Prior to the implementation of the principle of memory compaction in the kernel, lumpy reclaim was used for defragmentation. However, in version 3.10 (currently the most common), this functionality was removed. If you are interested in learning more about this technique, you can read the materials that I cited in the article A brief history of defragmentation.
Here I will immediately move on to the topic of memory compaction.
▍Introduction of the algorithm
The Memory Compaction article on LWN.net details the algorithmic principle of memory compaction. As a simple example, we can take the following fragmented zone:
Small fragmented memory area - LWN.netWhite blocks are free pages and red blocks are allocated pages. The compaction of memory in relation to this zone is divided into three main steps:
1. Scanning the zone from left to right in search of red pages with type
MIGRATE_MOVABLE
.
Search for moving pages2. At the same time, scan the area from right to left to search for free pages.
Search for free pages3. Shift the moved pages under the free ones to create a continuous section of free space.
Compacted memory zoneThe principle itself seems relatively simple, and the kernel also provides /proc/sys/vm/compact_memory
to start compacting the memory manually.
![]()
However, as mentioned at the beginning of this article, memory compaction in practice turns out to be particularly effective - at least not for the most common v3.10 - regardless of manual or automatic start. In view of the associated computational load, this operation, on the contrary, leads to the formation of a bottleneck.
Although the community members did not abandon this idea and continued to optimize it. For example, the kcompactd tool was later added to the v4.6 kernel, and direct compaction was made more specific in v4.8.
▍When memory compaction is performed
In the v3.10 kernel, this operation is performed in any of the following situations:
- Call thread
kswapd
to balance zones after a failed top-level allocation. - Call thread
khugepaged
to merge small chunks of memory into large pages. - Activate memory compaction manually via interface
/proc
.
The system resorts to immediate memory reclaiming, including the handling of Transparent Huge Pages (THP) failure exceptions, to meet the requirement to allocate higher-level memory.
THP functionality slows down performance, so it is recommended to disable this option. I will not analyze this nuance here and will focus mainly on the process of memory allocation.
Slow path memory allocation
If no available pages are found in the allocator lists during allocation, the following occurs: limit.
kswapd
thread to return the pages asynchronously and retry the allocation, also using the lower limit as the threshold. 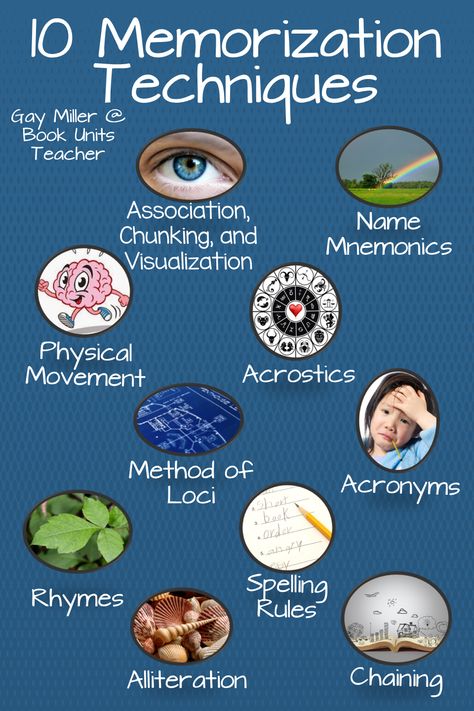
The steps listed are only a simplified description of the actual flow. In reality, it is more complicated and differs depending on the level of requested memory and allocation flags.
As for the direct return, it is performed only in case of a serious lack of memory, and also due to its fragmentation in practical scenarios. At certain times, both of these situations can occur simultaneously.
▍Memory compaction analysis
Delay Quantification
As discussed in the previous section, when memory is allocated, the kernel can reclaim or compact it. To make it easier to quantify the latency caused by direct memory reclaiming and compacting, I added two tools to the BCC project, drsnoop and compactsnoop.
They are both based on kernel events and well documented, but I do want to clarify one thing: to reduce the cost of introducing Berkeley Packet Filters (BPFs), these tools intercept the delay of each respective event. Therefore, it can be seen from the output that each memory request has multiple latency results.
The reason for this one-to-many relationship is that for older versions of the kernel like v3.10 it is not clear how many times the kernel will try to reclaim memory over the slow path. This uncertainty also causes OOM Killer to start running too early or too late. As a result, most tasks on the server are suspended for a long time.
After the implementation of the patch mm: fixed 100% CPU kswapd busyloop on unreclaimable nodes in v4.12, the maximum number of direct memory reclaim operations was limited to 16. Let's assume that the average delay of such an operation is 10 ms. (Pruning active or inactive LRU chain tables is costly on modern servers with several hundred gigabytes of RAM. Additional latency is also imposed if the server has to wait for a dirty page writeback.)
If a thread asks for a page allocator and after one immediate return receives empty memory, the return delay increases to 10 ms. If the kernel had to perform 16 such operations to return enough memory, the total latency for the entire allocation process would be already 160 ms, causing a serious performance hit.
Fragmentation Index View
Let's go back to memory compaction. The basic logic of this process is divided into four steps:
- Determine if a memory area is suitable for compaction.
- Sets the frame number of the start page to be scanned.
- Isolate pages of type
MIGRATE_MOVABLE
. - Move pages of type
MIGRATE_MOVABLE
to the top of the zone.
If, after one move, the zone still needs compaction, the core repeats this cycle three to four times until compaction is complete. This operation consumes a lot of processor resources, and therefore, when monitoring, you can often see its full load.
Okay, so how does the kernel determine if a zone is suitable for compacting memory?
If you use interface /proc/sys/vm/compact_memory
to force compaction of a zone's memory, then the kernel does not need to determine its suitability for this procedure.
If compaction is enabled automatically, the kernel calculates a fragmentation index of the requested level, determining whether there is enough memory left in the zone for compaction.
The closer the resulting index is to 0, the higher the probability of this operation failing due to lack of memory. This means that in such a case, a memory return operation is more suitable. An index approaching 1,000 increases the likelihood of an allocation failure due to excessive external fragmentation. Therefore, memory reclaiming rather than compaction should also be used in this situation.
The choice of the kernel in favor of compacting or returning memory is determined by the external fragmentation threshold, which can be viewed through the interface /proc/sys/vm/extfrag_threshold
.
You can also view the fragmentation index directly with cat /sys/kernel/debug/extfrag/extfrag_index
. Note that the results below are divided by 1,000:
Pros and cons0008 /proc , or with kernel event-based tools ( drsnoop
and compactsnoop
), but each has its own strengths and weaknesses.
Interface monitoring is easy to use, but it will not quantify latency results and the sampling period will be very long. Kernel-based tools, in turn, solve these problems, but in this case, you need to have a solid understanding of how the kernel subsystems work, and besides, there are certain requirements for its client version.
In fact, these methods complement each other well. Using both of them, you can fully analyze memory compaction.
Anti-fragmentation
The kernel has special mechanisms for working with slow backend devices. For example, it implements a second chance page replacement method, as well as a preset range based on the LRU algorithm, and there is no way to limit the allocation of part of the memory for the page cache ( page cache
). Some companies have customized the kernel to fit their needs to limit the page cache, and have even offered to implement these versions to the community, but they have been rejected. I think the reason is that this functionality causes a number of problems like preset working settings.
In this regard, in order to reduce the frequency of memory return operations and in order to combat fragmentation, it would be a good decision to increase vm.
(up to 5% of total memory). This will indirectly limit the share of the page cache in scenarios with a large number of I / O operations and in cases where the machine has more than 100GB of memory installed. min_free_kbytes
Although increasing vm.min_free_kbytes
results in some memory overhead, the overhead is negligible. For example, if the server's storage is 256GB and you set vm.min_free_kbytes
to 4G, that would only be 1.5% of the total space.
The community, of course, also noticed this memory cost, so a patch was added in v4.6 to optimize it accordingly.
Alternatively, can be done at the right time drop cache
, but this can lead to fluctuations in application performance.
Conclusion
At the beginning of the article, I briefly explained why external fragmentation affects performance and talked about the efforts made by the community in terms of defragmentation, after which I talked about the basic principles of defragmentation used in the v3.